
Clin Exp Dent Res. 2024 Nov 26;10(6):e70035. doi: 10.1002/cre2.70035
Mapping the Use of Artificial Intelligence–Based Image Analysis for Clinical Decision‐Making in Dentistry: A Scoping Review
On this page
ABSTRACT
Objectives
Artificial intelligence (AI) is an emerging field in dentistry. AI is gradually being integrated into dentistry to improve clinical dental practice. The aims of this scoping review were to investigate the application of AI in image analysis for decision‐making in clinical dentistry and identify trends and research gaps in the current literature.
Material and Methods
This review followed the guidelines provided by the Preferred Reporting Items for Systematic Reviews and Meta‐Analyses Extension for Scoping Reviews (PRISMA‐ScR). An electronic literature search was performed through PubMed and Scopus. After removing duplicates, a preliminary screening based on titles and abstracts was performed. A full‐text review and analysis were performed according to predefined inclusion criteria, and data were extracted from eligible articles.
Results
Of the 1334 articles returned, 276 met the inclusion criteria (consisting of 601,122 images in total) and were included in the qualitative synthesis. Most of the included studies utilized convolutional neural networks (CNNs) on dental radiographs such as orthopantomograms (OPGs) and intraoral radiographs (bitewings and periapicals). AI was applied across all fields of dentistry ‐ particularly oral medicine, oral surgery, and orthodontics ‐ for direct clinical inference and segmentation. AI‐based image analysis was use in several components of the clinical decision‐making process, including diagnosis, detection or classification, prediction, and management.
Conclusions
A variety of machine learning and deep learning techniques are being used for dental image analysis to assist clinicians in making accurate diagnoses and choosing appropriate interventions in a timely manner.
Keywords: artificial intelligence, convolutional neural networks, dentistry, image analysis
1. Introduction
In the modern era of rapid technological development, artificial intelligence (AI) has begun to permeate nearly every field of work. At its core, AI aspires to mimic the human brain, identifying patterns and understanding relationships between attributes and variables. Developments in AI have given rise to well‐established models that can exemplify traits such as learning, critical thinking, and decision‐making (Lingam et al. 2022). The most general and superficial classification under the broader AI umbrella term is shallow machine learning (Janiesch, Zschech, and Heinrich 2021). Algorithms in this classification are derived from decision trees or statistical models, which commonly involve linear and logistic regressions, Naive Bayes, and clustering. Taking a deeper step into the realm of machine learning, there is the introduction of artificial neural networks (ANNs). The networks consist of one or two layers of processing units, similar to artificial neurons. These neural units interact and continuously adjust as the network is trained and refined by researchers inputting data sets. In contrast, deep learning consists of many hidden layers embedded within the network architecture between the input and output levels. The additional depth allows for advanced operations and a greater capability to grasp complex patterns and intricacies. Among the frontiers of deep learning AI are convolutional neural networks (CNNs) and recurrent neural networks (RNNs). Ultimately, AI has an innate reliance on three major components: the computing capacity of hardware, the capability of algorithmic software, and an abundance of adequate data input (Ding et al. 2023).
Even fields distant from the forefront of computer sciences can reap the benefits, as they ride the tailwinds of constant digital advancements. To no exception, AI's integration into the field of dentistry already shows exciting improvements to current clinical practice (Ding et al. 2023). Deep learning has been applied to various facets of clinical care spanning disease detection, classification, prediction, prognosis, and management. Its integration as a clinical adjunct holds tremendous promise for advancing the goals of contemporary dentistry. Modern dentistry pillars include minimal intervention and nominalistic approaches in alignment with evidence‐supported treatment decisions to maximize long‐term oral health (Carounanidy and Sathyanarayanan 2009; Jingarwar, Bajwa, and Pathak 2014). Currently, AI's impact already extends to a wide array of dental specialties, ranging from interproximal caries detection in cariology (Bayraktar and Ayan 2022; García‐Cañas et al. 2023) to three‐dimensional jaw visualization and reconstruction for treatment planning in oral and maxillofacial surgery (OMFS) (Mima et al. 2022; Xu, Liu, and Zheng 2019).
Amidst the rapidly evolving landscape of AI technology, a multitude of proposed applications and algorithms have emerged. Therefore, the objective of this scoping review is to provide a comprehensive assessment and summary of the existing literature on AI‐based image analysis and its impact on decision‐making in clinical dentistry. This review aims to scope and illustrate the variety of existing models, their current utilization among different imaging modalities, research gaps, and emerging trends and frontiers within this applied discipline.
2. Methods
2.1. Search Strategy
This scoping review was conducted in accordance with the Preferred Reporting Items for Systematic Reviews and Meta‐Analyses Extension for Scoping Reviews (PRISMA‐ScR) guidelines (Tricco et al. 2018), as reported in Table S1. The following databases were searched:
1.PubMed
2.Scopus
Studies published before the month of June 2023 were included when searching databases, with no restrictions placed on the date of publication. Studies that were not published in English, studied non‐human populations, and all reviews were excluded via automatic database filtering tools. Furthermore, conference papers and non‐peer‐reviewed literature were manually excluded by reviewers.
The aim of this scoping review was to explore the incorporation of AI‐based image analysis into clinical decision‐making within dentistry. To obtain a broad initial range of studies, synonyms for, or terms associated with AI were included in the search string such as “convolution neural network,” “machine learning,” “deep learning,” and “support vector machine.” To focus our search strategy on image analysis, keywords such as “diagnosis” and “accuracy” were included; however, classification into specific imaging modalities was performed at a later screening stage.
The following search string was utilized to obtain the initial set of relevant studies on PubMed: ((“Artificial Intelligence”) OR (“AI”) OR (“Artificial neural network”) OR (“Conventional neural network”) OR (“Convolutional neural network”) OR (“Deep learning”) OR (“Machine learning”) OR (“Computational Intelligence”) OR (“Machine Intelligence”) OR (“Support vector machine”) OR (“Artificial Intelligence”[Mesh]) OR (“Deep Learning”[Mesh])) AND ((Dent*[Title/Abstract]) OR (Dentistry[Mesh]) OR (“teeth”) or (“tooth”) OR (“oral”)) AND ((“Diagnos*”) OR (“Decision‐making”) OR (“Computer‐aided diagnosis”) OR (“Clinical Decision‐Making” [Mesh]) OR (“Decision Making, Computer‐Assisted” [Mesh])) AND ((“Efficacy”) OR (“Accuracy”) OR (“Effectiveness”)). This search was modified when searching Scopus with the additional keyword combination: AND (EXCLUDE (DOCTYPE, “re”)) AND (LIMIT‐TO (LANGUAGE, “English”)) AND (LIMIT‐TO (EXACTKEYWORD, “Human”)).
To maintain a broad scope, studies in all fields and specialities of clinical dentistry (excluding forensic and teledentistry) were considered in this study. Reviewers cross‐checked and manually removed studies deemed irrelevant based on the AI's role in decision‐making, field of application and imaging modality.
2.2. Data Extraction
Relevant papers obtained from both databases were compiled, and duplicates were removed. The remaining papers were then divided between three pairs of reviewers and screened by titles and abstracts on the screening website Rayyan (https://www.rayyan.ai/). Studies were classified by dental field, imaging modality, and application of AI and included/excluded based on these categories.
Once all conflicts between reviewers were resolved via team discussion, the remaining included papers were extracted and imported into a data extraction table, where studies were categorized based on imaging modality, field of dentistry, AI's role in decision‐making, method of AI application, and type and classification of AI algorithms used. The role of AI in decision‐making was separated into three main categories: diagnosis/detection/classification, prediction, and management. In terms of AI application, algorithms with a direct clinical influence on decision‐making or those responsible for segmentation were included, whereas studies with AI utilized solely as a research technique with no clinical influence were excluded. Studies in all fields of dentistry were included, except for forensic dentistry, as these findings have more limited application in general clinical dentistry and the treatment of living patients.
Additional exclusion criteria included histology, in vitro methods, traditional Chinese medicine, and teledentistry, as these were deemed irrelevant to the “chairside” clinical dentistry focus of this review. Studies that involved no imaging input at all or utilized forms of imaging uncommon in clinical dental practice were also excluded. Additionally, any non‐peer‐reviewed papers or irretrievable full‐text studies were excluded (Table S2).
2.3. Data Charting
Once the final set of included studies was established, data collected from the data extraction table were categorized and presented in graphical form. The graphs illustrate the distribution of studies across various categories, including the role in decision‐making, dental field, application of AI, imaging modality, and AI algorithm. Additional graphs were included to display and compare the prevalence of imaging modalities within each dental field.
3. Results
3.1. Selection of Sources of Evidence
The PRISMA flowchart of the scoping review process is shown in Figure 1. The search strategy retrieved 1334 articles from two databases (PubMed and Scopus). Following the removal of 333 duplicates, title and abstract screening of 1001 articles was conducted. Full‐text screening of 381 articles was completed, of which 109 articles were excluded for reasons outlined in Figure 1. Finally, 276 articles were included in the qualitative synthesis. A summary of the relevant data from the included articles is presented in Table S1.
Figure 1.
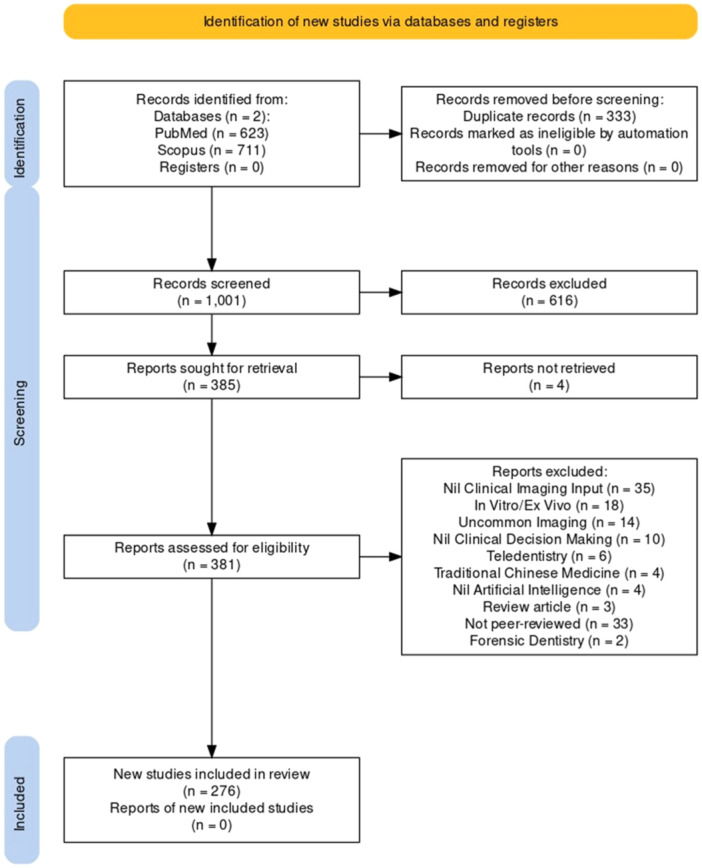
Preferred Reporting Items for Systematic Reviews and Meta‐Analyses Extension for Scoping Reviews (PRISMA‐ScR) flowchart.
3.2. Characteristics of Sources of Evidence
The majority of the included articles were published from 2019 onward, with the number of publications increasing each year (Figure S1). The sizes of the data sets ranged from 10 to 83,676 images, totaling 601,122 items.
3.2.1. Application of AI in Clinical Decision‐Making
The majority of articles discussed the use of AI to directly influence clinical decision‐making (n = 231, 84%), whereas some involved AI for segmentation of anatomical structures (n = 45, 16.3%) (Figure S2). AI‐based image analysis was most commonly used for diagnosis, detection, or classification (n = 233, 84.4%), followed by prediction (n = 30, 10.9%) and management (n = 13, 4.7%) (Figure S3).
Many articles discussed the use of AI across multiple fields of dentistry and hence were categorized into more than one area (n = 32, 12%). Of the 268 articles that evaluated AI application in diagnostic decision‐making, the greatest proportion was applied in the fields of oral medicine (n = 51, 19%), followed by OMFS (n = 44, 16%) and operative dentistry (n = 41, 15%) (Figure 2). Out of the 13 articles that assessed AI application in management decision‐making, the greatest proportion was applied in the fields of OMFS (n = 5, 38%), followed by orthodontics (n = 4, 31%) (Figure 2). From the 30 articles that analyzed predictive decision‐making with AI, the most common field of application was orthodontics (n = 11, 29%), followed by OMFS (n = 8, 21%) and oral medicine (n = 7, 18%) (Figure 2).
Figure 2.
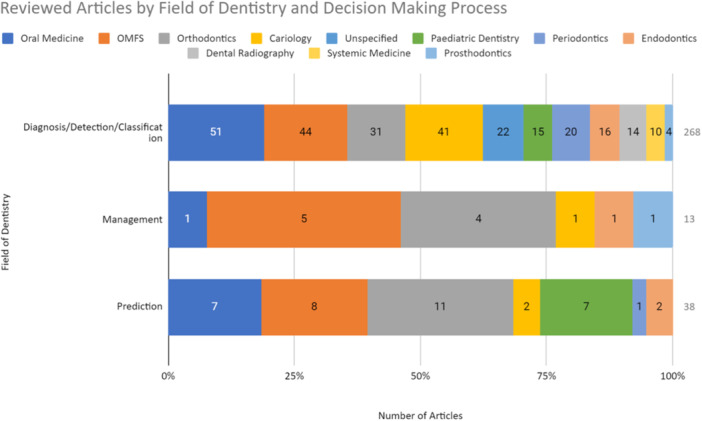
AI‐assisted decision‐making process and fields of dentistry involved in the reviewed articles. Many articles discussed the use of AI in multiple fields and were categorized as such; therefore, there is a discrepancy in the total number. OMFS, oral and maxillofacial surgery. Cariology (operative dentistry).
Similar to articles exploring AI applications in more than one field of dentistry, many models utilized more than a single imaging modality (n = 17, 6%). The input imaging modality most commonly evaluated as the input across all decision‐making categories was orthopantomograms (OPGs)—30% of diagnostic articles (n = 73), 27% of management articles (n = 4), and 33% of prediction‐based articles (n = 13) (Figure 3). Following OPG, AI‐assisted diagnostic decision‐making was assessed with intra‐oral radiographs (n = 49, 20%) and management and predictive‐based decision‐making with cephalograms (n = 1 and n = 5, respectively) (Figure 3A).
Figure 3.
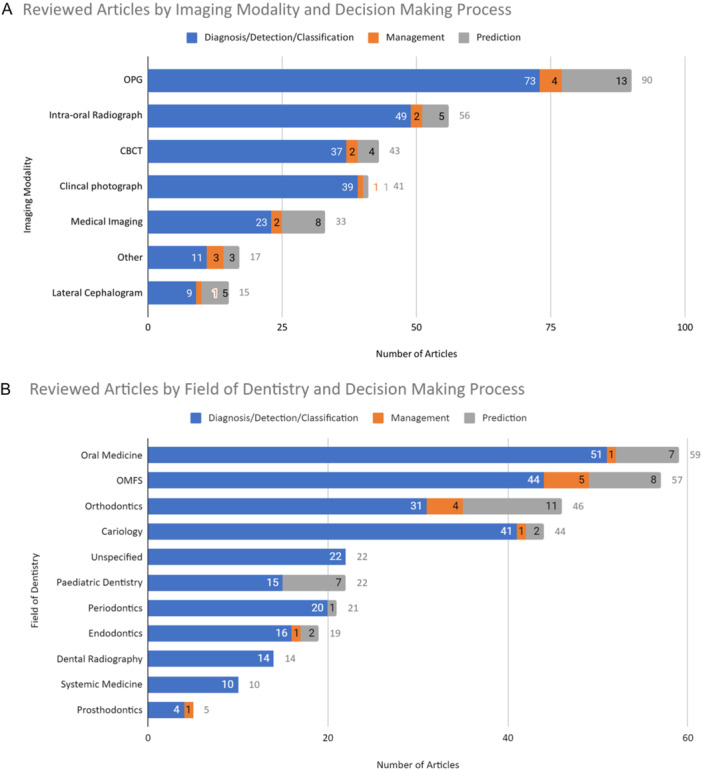
(A) The distribution of reviewed articles by imaging modality utilized as the input for AI analysis and decision‐making process. Abbreviations: CBCT, cone‐beam computed tomography; OPG, orthopantomogram. Medical imaging includes magnetic resonance imaging (MRI), computed tomography (CT), and ultrasound. Others include optical coherence tomography (OCT), hand‐wrist radiograph, phone images, video analysis, digital infrared thermal imaging, and 3D dental scanner. (B) The distribution of reviewed articles by the field of dentistry and the decision‐making process involved. OMFS, oral and maxillofacial surgery.
3.2.2. Fields of Dentistry
AI‐based image analysis was applied most commonly in the following fields of dentistry: oral medicine (n = 59, 19%), OMFS (n = 57, 16%), orthodontics (n = 46, 12%), and operative dentistry (n = 44, 15%) (Figure 3B).
The field of dentistry with the greatest proportion of articles reviewing AI‐assisted diagnostic decision‐making was for the detection of carious lesions (n = 41, 93%). Prosthodontics was the field with the greatest proportion of articles evaluating AI for management decisions (n = 1, 20%) (Figure 3B). Notably, the field with the highest proportion of AI applications in predictive decisions was pediatric dentistry (n = 7, 32%) where AI was used for dental age estimation and orthodontics (n = 11, 24%) for the prediction of growth and development using cephalograms.
Eighty‐six percent of oral medicine–related articles (n = 51) discussed AI for diagnostic decision‐making, particularly for the detection of oral cancer and associated lymph node metastases and odontogenic cystic lesions. Within the OMFS field (n = 57) for the detection of anatomical structures (n = 44) (Figure 3B), AI was most commonly used for the detection of impacted third molars and their proximity to the inferior alveolar nerve, or the detection of mandibular fractures.
3.2.3. Imaging Modalities
Imaging modalities most commonly used for AI analysis were OPGs (n = 90, 33%), intra‐oral radiographs (n = 56, 20%), cone‐beam computed tomography (CBCTs) (n = 43, 15%), and clinical photographs (n = 41, 14%) (Figure 3A). The imaging modality with the greatest proportion of articles assessing AI‐assisted diagnostic decisions was clinical photographs (n = 39, 95%) for the detection of carious lesions and OSCC, whereas cephalogram was the imaging modality with the greatest proportion of prediction‐based articles (n = 5, 33%).
3.2.4. AI Algorithm
Figure 4 demonstrates the classification of AI models assessed in the reviewed articles. The majority of articles demonstrated the use of ANN/multilayer perceptron (MLP) (n = 258, 93%) (further details in Figure S4) to address clinical problems, of which the majority used CNN models (n = 204, 79%) (further details in Figure S5). The most commonly utilized CNN model was ResNet (n = 55, 27%), followed by U‐net (n = 31, 15%) and R‐CNN (n = 31, 15%) (Figure S6).
Figure 4.
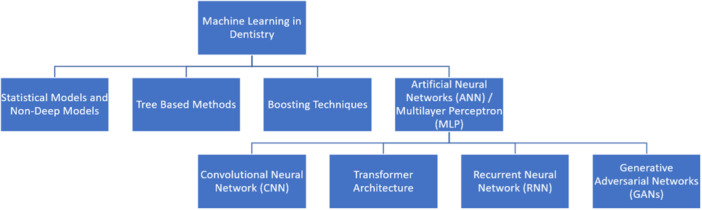
The classification of machine learning algorithms demonstrated in the reviewed articles.
3.2.5. Summary of Largest Data sets
Hernandez et al. (2021) conducted a study with the largest data set to date, comprising 83,676 head and neck CT slices from 549 patients. Their CNN system achieved high accuracy in detecting dental artifacts on a slice‐by‐slice basis, demonstrating the potential use of AI in improving diagnostic precision. Similarly, Kearney et al. (2022) utilized a large data set of 80,326 images for training, with images for validation and testing, to develop a tool for determining clinical attachment loss (CAL) in the diagnosis of periodontal disease. Their model accurately calculated CAL within 1 mm of clinician‐determined standards, highlighting its clinical relevance. Additionally, Fu et al. (2020) developed a CNN model using 44,409 images, with both external and internal validation, and found that the model's performance in detecting oral cancer was comparable to that of oral cancer specialists. Collectively, across all 276 articles included in this qualitative synthesis, approximately 600,000 images were used in various phases of training AI systems, validation processes, and testing.
4. Discussion
In this scoping review, we systematically examined the implementation of AI‐based image analysis for clinical decision‐making in dentistry. The review sought to provide a comprehensive overview of the current research present in this rapidly emerging field by analyzing a diverse range of studies. Next, we summarized the main themes and trends that emerged from the literature, address existing research gaps, and consider the potential implications of AI‐based image analysis for dental practitioners in clinical decision‐making.
4.1. Summary of Evidence
This review identified 276 primary studies addressing AI‐based image analysis, with the majority of the articles emerging after 2019. This reflects the rapidly evolving nature of AI in an attempt to aid clinical decision‐making.
The most frequently researched categories were oral medicine, OMFS, and operative dentistry, where the technology was used for neoplastic disease, anatomical and structural interpretations, and detection of caries. For all fields of dentistry, the specific use of AI has been primarily in the detection/diagnosis/classification. This is likely due to improving the efficiency of identifying issues so that dental clinicians can act promptly to address them for patients. One such example is in oral medicine where it can be used to identify cancers and metastatic disease, which necessitates early identification and treatment. Currently, such diagnoses require specialist input; however, with such technology, it may assist general dentists to flag findings and expedite the treatment pathway for patients. Interestingly, the greatest proportion of predictive AI studies was in orthodontics (n = 8, 21%) and OMFS (n = 7, 18%). In these specialties, the goal is not only addressing patient concerns in the chair but attempting to predict outcomes and trajectories of treating interventions. In the field of orthodontics, AI can be utilized to predict the need for orthodontic interventions through the analysis of imaging techniques such as lateral cephalograms. Furthermore, predictive AI in orthodontics has been utilized to assess the need for orthognathic surgery solely based on facial photographs.
In terms of imaging, OPGs (n = 90, 33%) and intra‐oral radiographs (n = 56, 20%) were the most common. Clinicians are well‐versed in acquiring and interpreting OPGs as they are commonly performed for patients and are readily available. OPGs are clinically relevant to many different specialties such as OMFS and periodontics, making them a useful imaging modality for AI algorithms. In contrast, intra‐oral radiographs (bitewings and periapicals) are imperative for caries detection and diagnosis, which is the reason why over 19% of articles used this modality for their algorithm.
The majority of studies used neural networks to generate AI models. The most frequently reported type of neural network was CNN (n = 204, 74%) that mimics the visual processing capabilities of the human brain, hence the name. These models excel at extracting features from images via hierarchical feature extraction and are capable of learning spatial invariance to recognize patterns and objects regardless of position or orientation within the image (Alzubaidi et al. 2021). This makes it a superior tool for dentistry where there can be a lot of variability in the imaging of patients.
4.2. Research Gaps
The use of AI in dentistry is still an emerging field, with limited application to clinical practice at this point. Some barriers include the need for large volumes of training data, inconsistency in data annotation, and population demographics. Although some studies had ample sample sizes in the tens of thousands, others were well below a hundred, which can impact the training and resulting performance of the algorithms. Although AI models are meant to learn independently from a data set, initially, they may require varying extents of annotation within subsets and training. Due to the requirement of large quantities of training data, there can be multiple annotators, introducing variability in identifying the necessary features for the algorithm to use. Another limitation in this scoping review and applicability to clinical practice in Australia is the lack of representation in population demographics—the majority of the studies were from mainly Asian countries such as South Korea and China. Although dental practice may be similar in these regions, the epidemiology and data used for training may not apply as closely to populations in Australia.
4.3. Implications and Future Applications
Clinical decision‐making in dentistry is a complex process as numerous aspects of dental examination rely on indirect measures. Therefore, dental clinicians are required to have profound knowledge with respect to basic principles and appropriate evaluation regarding diagnostic tests and published literature. Nevertheless, discrepancies often arise among experienced practitioners regarding clinical decision‐making. In the field of dentistry, a gold standard is defined as the benchmark with known results that is the best available under reasonable conditions (Cardoso et al. 2014). Introducing benchmarks through AI can be beneficial in facilitating dental practitioners, especially less experienced ones, to make more informed clinical decisions.
A diverse image modality can be processed by AI, which CT and MRI require knowledge of systemic medicine and can be a challenge for dental practitioners. AI‐assisted decision‐making has the potential to benefit dentists in making more informed diagnoses. However, there is currently no standardization with respect to the amount of data set to be trained per algorithm as well as demographics mentioned in the previous section, thus potentially challenging the accuracy and validity of clinical AI applications.
Segmentation plays a crucial role in processing data from imagery and has been applied widely including for the development of computer‐assisted diagnosis (CAD) using various different modalities of radiological images, such as cephalograms, OPGs and CTs. The mechanism involves processing clusters of images into coherent sub‐regions based on extracted features, for instance, texture attributes (Seo et al. 2020). Forty‐five articles evaluating AI segmentation on different imagery modalities in dentistry were included in this review. Although the technology is not directly contributing to decision‐making, the papers investigating segmentation showed great proof‐of‐concept potential and preliminary work toward processes ranging from automatic landmark identification to tooth segmentation without manual labeling.
AI's potential in dentistry has the ability to extend beyond AI‐assisted diagnosis during appointments. For example, six papers revealed novel AI‐driven detection of oral lesions at home or in remote settings. They are excluded from this review as the application did not directly involve in‐chair practice. However, it is important to encourage research in these areas as it could improve early detection, prevent diagnostic delays, and encourage patient–clinician communication. Another study by Schwendicke et al. (2022) evaluated the cost‐effectiveness of AI diagnosis. Although there is currently no formal significant evidence proving that AI diagnosis is more cost‐effective, it is postulated that AI applications can be advantageous in reducing practitioner workload and chairside time. These factors may benefit both clinicians and patients as overtreatment and misdiagnosis are minimized, and resources become better allocated.
Despite AI‐assisted decision‐making showing great potential, it is important to discuss the ethical issues involved. AI training and application require the input of patient data, meaning there should be regulations introduced in relation to privacy and data security. The use of patient data as part of AI training suggests that patient information will continuously be applied as part of the algorithm; hence, patients should be informed appropriately. Human oversight and associated liability and accountability can be a challenge in AI‐assisted decision‐making as a large portion of the articles reviewed were designed to assist less‐experienced practitioners. This raises questions such as whether a less‐experienced clinician is able to correct the error made by AI, and if AI concludes an incorrect diagnosis, who will be held liable for the potential damages to the patient.
5. Conclusions
This review demonstrated the diverse use of AI in dentistry, especially in the past 5 years. AI is progressively gaining a foothold in clinical dentistry and is used for a wide array of purposes across all fields of dentistry. Current studies adopted a variety of machine learning and deep learning techniques for analysis across several dental image modalities to assist in clinical decision‐making and in efforts toward achieving better patient outcomes. Most studies provided initial proof of concept and advocated for both further development and future implementation of AI in dentistry. Therefore, systematic reviews and meta‐analyses of the current literature should be performed to critically appraise the quality and validity of these studies to objectively evaluate the reliability of AI use in dental practice. In addition, future studies in clinical settings across different countries and the standardization of training protocol objectives should be conducted to fill in existing research gaps.
Author Contributions
Investigation, formal analysis, data curation, writing–original draft: Wei Chen, Monisha Dhawan, Jonathan Liu, Damie Ing, Kruti Mehta, and Daniel Tran. Conceptualization, writing–review and editing, supervision: Daniel Lawrence and Max Ganhewa. Conceptualization, methodology, writing–review and editing, supervision, project administration: Nicola Cirillo.
Ethics Statement
This is a review article and did not require ethical clearance.
Conflicts of Interest
M.G. is the founder and CEO of CoTreat Pty Ltd, Melbourne, Victoria, Australia.
Supporting information
Supporting information.
CRE2-10-e70035-s001.docx (358KB, docx)
Acknowledgments
The authors would like to acknowledge the support of the Melbourne Dental School at the University of Melbourne for making the necessary resources available. This study received no external funding. Open access publishing facilitated by the University of Melbourne, as part of the Wiley ‐ University of Melbourne agreement via the Council of Australian University Librarians.
Data Availability Statement
The data that support the findings of this study are available in the Supporting Information of this article.
References
*References marked with asterisk are cited in Supplementary Table S1.
- *Agarwal, P., Yadav A., Mathur P., Pal V., and Chakrabarty A.. 2022. “BID‐Net: An Automated System for Bone Invasion Detection Occurring at Stage T4 in Oral Squamous Carcinoma Using Deep Learning.” Computational Intelligence and Neuroscience2022: 4357088. 10.1155/2022/4357088. [DOI] [PMC free article] [PubMed] [Google Scholar] [Retracted]
- *Ahn, Y., Hwang J. J., Jung Y.‐H., Jeong T., and Shin J.. 2021. “Automated Mesiodens Classification System Using Deep Learning on Panoramic Radiographs of Children.” Diagnostics11, no. 8: 1477. 10.3390/diagnostics11081477. [DOI] [PMC free article] [PubMed] [Google Scholar]
- *Alalharith, D. M., Alharthi H. M., Alghamdi W. M., et al. 2020. “A Deep Learning‐Based Approach for the Detection of Early Signs of Gingivitis in Orthodontic Patients Using Faster Region‐Based Convolutional Neural Networks.” International Journal of Environmental Research and Public Health17, no. 22: 8447. 10.3390/ijerph17228447. [DOI] [PMC free article] [PubMed] [Google Scholar]
- *Al‐Ghamdi, A. S. A.‐M., Ragab M., Alghamdi S. A., Asseri A. H., Mansour R. F., and Koundal D.. 2022. “Detection of Dental Diseases Through X‐Ray Images Using Neural Search Architecture Network.” Computational Intelligence and Neuroscience2022: 1–7. 10.1155/2022/3500552. [DOI] [PMC free article] [PubMed] [Google Scholar]
- *Aljabri, M., Aljameel S. S., Min‐Allah N., et al. 2022. “Canine Impaction Classification From Panoramic Dental Radiographic Images Using Deep Learning Models.” Informatics in Medicine Unlocked30: 100918. 10.1016/j.imu.2022.100918. [DOI] [Google Scholar]
- *Al‐Ma'aitah, M., and AlZubi A. A.. 2018. “Enhanced Computational Model for Gravitational Search Optimized Echo State Neural Networks Based Oral Cancer Detection.” Journal of Medical Systems42, no. 11: 205. 10.1007/s10916-018-1052-0. [DOI] [PubMed] [Google Scholar]
- *Alotaibi, G., Awawdeh M., Farook F. F., Aljohani M., Aldhafiri R. M., and Aldhoayan M.. 2022. “Artificial Intelligence (Ai) Diagnostic Tools: Utilizing a Convolutional Neural Network (CNN) to Assess Periodontal Bone Level Radiographically—A Retrospective Study.” BMC Oral Health22, no. 1: 399. 10.1186/s12903-022-02436-3. [DOI] [PMC free article] [PubMed] [Google Scholar]
- Alzubaidi, L., Zhang J., Humaidi A. J., et al. 2021. “Review of Deep Learning: Concepts, CNN Architectures, Challenges, Applications, Future Directions.” Journal of Big Data8, no. 1: 53. 10.1186/s40537-021-00444-8. [DOI] [PMC free article] [PubMed] [Google Scholar]
- *Andrade, K. M., Silva B. P. M., de Oliveira L. R., and Cury P. R.. 2023. “Automatic Dental Biofilm Detection Based on Deep Learning.” Journal of Clinical Periodontology50, no. 5: 571–581. 10.1111/jcpe.13774. [DOI] [PubMed] [Google Scholar]
- *De Angelis, F., Pranno N., Franchina A., et al. 2022. “Artificial Intelligence: A New Diagnostic Software in Dentistry: A Preliminary Performance Diagnostic Study.” International Journal of Environmental Research and Public Health19, no. 3: 1728. 10.3390/ijerph19031728. [DOI] [PMC free article] [PubMed] [Google Scholar]
- *De Araujo Faria, V., Azimbagirad M., Viani Arruda G., et al. 2021. “Prediction of Radiation‐Related Dental Caries Through Pyradiomics Features and Artificial Neural Network on Panoramic Radiography.” Journal of Digital Imaging34, no. 5: 1237–1248. 10.1007/s10278-021-00487-6. [DOI] [PMC free article] [PubMed] [Google Scholar]
- *Ariji, Y., Fukuda M., Kise Y., et al. 2019. “Contrast‐Enhanced Computed Tomography Image Assessment of Cervical Lymph Node Metastasis in Patients With Oral Cancer by Using a Deep Learning System of Artificial Intelligence.” Oral Surgery, Oral Medicine, Oral Pathology and Oral Radiology127, no. 5: 458–463. 10.1016/j.oooo.2018.10.002. [DOI] [PubMed] [Google Scholar]
- *Ariji, Y., Fukuda M., Nozawa M., et al. 2021. “Automatic Detection of Cervical Lymph Nodes in Patients with Oral Squamous Cell Carcinoma Using a Deep Learning Technique: a Preliminary Study.” Oral Radiology37, no. 2: 290–296. 10.1007/s11282-020-00449-8. [DOI] [PubMed] [Google Scholar]
- *Ariji, Y., Sugita Y., Nagao T., et al. 2020. “Ct Evaluation of Extranodal Extension of Cervical Lymph Node Metastases in Patients With Oral Squamous Cell Carcinoma Using Deep Learning Classification.” Oral Radiology36, no. 2: 148–155. 10.1007/s11282-019-00391-4. [DOI] [PubMed] [Google Scholar]
- *Askar, H., Krois J., Rohrer C., et al. 2021. “Detecting White Spot Lesions on Dental Photography Using Deep Learning: A Pilot Study.” Journal of Dentistry107: 103615. 10.1016/j.jdent.2021.103615. [DOI] [PubMed] [Google Scholar]
- *Awais, M., Ghayvat H., Krishnan Pandarathodiyil A., et al. 2020. “Healthcare Professional in the Loop (HPIL): Classification of Standard and Oral Cancer‐Causing Anomalous Regions of Oral Cavity Using Textural Analysis Technique in Autofluorescence Imaging.” Sensors20, no. 20: 5780. 10.3390/s20205780. [DOI] [PMC free article] [PubMed] [Google Scholar]
- *Ayidh Alqahtani, K., Jacobs R., Smolders A., et al. 2023. “Deep Convolutional Neural Network‐Based Automated Segmentation and Classification of Teeth With Orthodontic Brackets on Cone‐Beam Computed‐Tomographic Images: A Validation Study.” European Journal of Orthodontics45, no. 2: 169–174. 10.1093/ejo/cjac047. [DOI] [PubMed] [Google Scholar]
- *Banar, N., Bertels J., Laurent F., et al. 2020. “Towards Fully Automated Third Molar Development Staging in Panoramic Radiographs.” International Journal of Legal Medicine134, no. 5: 1831–1841. 10.1007/s00414-020-02283-3. [DOI] [PubMed] [Google Scholar]
- Bayraktar, Y., and Ayan E.. 2022. “Diagnosis of Interproximal Caries Lesions With Deep Convolutional Neural Network in Digital Bitewing Radiographs.” Clinical Oral Investigations26, no. 1: 623–632. 10.1007/s00784-021-04040-1. [DOI] [PMC free article] [PubMed] [Google Scholar]
- *Berdouses, E. D., Koutsouri G. D., Tripoliti E. E., Matsopoulos G. K., Oulis C. J., and Fotiadis D. I.. 2015. “A Computer‐Aided Automated Methodology for the Detection and Classification of Occlusal Caries From Photographic Color Images.” Computers in Biology and Medicine62: 119–135. 10.1016/j.compbiomed.2015.04.016. [DOI] [PubMed] [Google Scholar]
- *Bharathi, M., Prasad D., Venkatakrishnamoorthy T., and Dharani M.. 2022. “Diabetes Diagnostic Method Based on Tongue Image Classification Using Machine Learning Algorithms.” Journal of Pharmaceutical Negative Results13, no. 4: 1247–1250. 10.47750/pnr.2022.13.04.174. [DOI] [Google Scholar]
- *Bhattacharjee, N.2022. “Automated Dental Cavity Detection System Using Deep Learning and Explainable AI.” AMIA Joint Summits on Translational Science Proceedings. AMIA Joint Summits on Translational Science2022: 140–148. [PMC free article] [PubMed] [Google Scholar]
- *Bispo, M. S., Pierre Júnior M. L. G. Q., A. L.Apolinário, Jr., et al. 2021. “Computer Tomographic Differential Diagnosis of Ameloblastoma and Odontogenic Keratocyst: Classification Using a Convolutional Neural Network.” Dento Maxillo Facial Radiology50, no. 7: 20210002. 10.1259/dmfr.20210002. [DOI] [PMC free article] [PubMed] [Google Scholar]
- *Buyuk, C., Akkaya N., Arsan B., Unsal G., Aksoy S., and Orhan K.. 2022. “A Fused Deep Learning Architecture for the Detection of the Relationship Between the Mandibular Third Molar and the Mandibular Canal.” Diagnostics12, no. 8: 2018. 10.3390/diagnostics12082018. [DOI] [PMC free article] [PubMed] [Google Scholar]
- *Buyuk, C., Arican Alpay B., and Er F.. 2023. “Detection of the Separated Root Canal Instrument on Panoramic Radiograph: A Comparison of LSTM and CNN Deep Learning Methods.” Dentomaxillofacial Radiology52, no. 3: 20220209. 10.1259/dmfr.20220209. [DOI] [PMC free article] [PubMed] [Google Scholar]
- *Camalan, S., Mahmood H., Binol H., et al. 2021. “Convolutional Neural Network‐Based Clinical Predictors of Oral Dysplasia: Class Activation Map Analysis of Deep Learning Results.” Cancers13, no. 6: 1291. 10.3390/cancers13061291. [DOI] [PMC free article] [PubMed] [Google Scholar]
- *Cantu, A. G., Gehrung S., Krois J., et al. 2020. “Detecting Caries Lesions of Different Radiographic Extension on Bitewings Using Deep Learning.” Journal of Dentistry100: 103425. 10.1016/j.jdent.2020.103425. [DOI] [PubMed] [Google Scholar]
- Cardoso, J. R., Pereira L. M., Iversen M. D., and Ramos A. L.. 2014. “What Is Gold Standard and What Is Ground Truth?” Dental Press Journal of Orthodontics19, no. 5: 27–30. 10.1590/2176-9451.19.5.027-030.ebo. [DOI] [PMC free article] [PubMed] [Google Scholar]
- *Carmody, D. P., McGrath S. P., Dunn S. M., Van Der Stelt P. F., and Schouten E.. 2001. “Machine Classification of Dental Images With Visual Search.” Academic Radiology8, no. 12: 1239–1246. 10.1016/S1076-6332(03)80706-7. [DOI] [PubMed] [Google Scholar]
- Carounanidy, U., and Sathyanarayanan R.. 2009. “Dental Caries: A Complete Changeover (Part Ii)‐Changeover in the Diagnosis and Prognosis.” Journal of Conservative Dentistry12, no. 3: 87–100. 10.4103/0972-0707.57631. [DOI] [PMC free article] [PubMed] [Google Scholar]
- *Casalegno, F., Newton T., Daher R., et al. 2019. “Caries Detection With Near‐Infrared Transillumination Using Deep Learning.” Journal of Dental Research98, no. 11: 1227–1233. 10.1177/0022034519871884. [DOI] [PMC free article] [PubMed] [Google Scholar]
- *Celik, M. E.2022. “Deep Learning Based Detection Tool for Impacted Mandibular Third Molar Teeth.” Diagnostics12, no. 4: 942. 10.3390/diagnostics12040942. [DOI] [PMC free article] [PubMed] [Google Scholar]
- *Chai, Z.‐K., Mao L., Chen H., et al. 2022. “Improved Diagnostic Accuracy of Ameloblastoma and Odontogenic Keratocyst on Cone‐Beam CT by Artificial Intelligence.” Frontiers in Oncology11: 793417. 10.3389/fonc.2021.793417. [DOI] [PMC free article] [PubMed] [Google Scholar]
- *Chandrashekar, G., AlQarni S., Bumann E. E., and Lee Y.. 2022. “Collaborative Deep Learning Model for Tooth Segmentation and Identification Using Panoramic Radiographs.” Computers in Biology and Medicine148: 105829. 10.1016/j.compbiomed.2022.105829. [DOI] [PubMed] [Google Scholar]
- *Chang, H.‐J., Lee S.‐J., Yong T.‐H., et al. 2020. “Deep Learning Hybrid Method to Automatically Diagnose Periodontal Bone Loss and Stage Periodontitis.” Scientific Reports10, no. 1: 7531. 10.1038/s41598-020-64509-z. [DOI] [PMC free article] [PubMed] [Google Scholar]
- *Chang, J., Chang M.‐F., Angelov N., et al. 2022. “Application of Deep Machine Learning for the Radiographic Diagnosis of Periodontitis.” Clinical Oral Investigations26, no. 11: 6629–6637. 10.1007/s00784-022-04617-4. [DOI] [PubMed] [Google Scholar]
- *Chen, H., Li H., Zhao Y., Zhao J., and Wang Y.. 2021. “Dental Disease Detection on Periapical Radiographs Based on Deep Convolutional Neural Networks.” International Journal of Computer Assisted Radiology and Surgery16, no. 4: 649–661. 10.1007/s11548-021-02319-y. [DOI] [PubMed] [Google Scholar]
- *Chen, Q., Huang J., Salehi H. S., et al. 2021. “Hierarchical CNN‐Based Occlusal Surface Morphology Analysis for Classifying Posterior Tooth Type Using Augmented Images From 3D Dental Surface Models.” Computer Methods and Programs in Biomedicine208: 106295. 10.1016/j.cmpb.2021.106295. [DOI] [PubMed] [Google Scholar]
- *Chen, X., Guo J., Ye J., Zhang M., and Liang Y.. 2023. “Detection of Proximal Caries Lesions on Bitewing Radiographs Using Deep Learning Method.” Caries Research56, no. 5: 455–463. 10.1159/000527418. [DOI] [PMC free article] [PubMed] [Google Scholar]
- *Cheng, B., and Wang W.. 2019. “Dental Hard Tissue Morphological Segmentation With Sparse Representation‐Based Classifier.” Medical & Biological Engineering & Computing57, no. 8: 1629–1643. 10.1007/s11517-019-01985-0. [DOI] [PubMed] [Google Scholar]
- *Chifor, R., Hotoleanu M., Marita T., et al. 2022. “Automatic Segmentation of Periodontal Tissue Ultrasound Images With Artificial Intelligence: A Novel Method for Improving Dataset Quality.” Sensors22, no. 19: 7101. 10.3390/s22197101. [DOI] [PMC free article] [PubMed] [Google Scholar]
- *Choi, E., Kim D., Lee J. Y., and Park H. K.. 2021. “Artificial Intelligence in Detecting Temporomandibular Joint Osteoarthritis on Orthopantomogram.” Scientific Reports11, no. 1: 10246. 10.1038/s41598-021-89742-y. [DOI] [PMC free article] [PubMed] [Google Scholar]
- *Choi, E., Lee S., Jeong E., et al. 2022. “Artificial Intelligence in Positioning Between Mandibular Third Molar and Inferior Alveolar Nerve on Panoramic Radiography.” Scientific Reports12, no. 1: 2456. 10.1038/s41598-022-06483-2. [DOI] [PMC free article] [PubMed] [Google Scholar]
- *Chung, E.‐J., Yang B.‐E., Park I.‐Y., et al. 2022. “Effectiveness of Cone‐Beam Computed Tomography‐Generated Cephalograms Using Artificial Intelligence Cephalometric Analysis.” Scientific Reports12, no. 1: 20585. 10.1038/s41598-022-25215-0. [DOI] [PMC free article] [PubMed] [Google Scholar]
- *Chung, M., Lee J., Park S., et al. 2021. “Individual Tooth Detection and Identification From Dental Panoramic X‐Ray Images via Point‐Wise Localization and Distance Regularization.” Artificial Intelligence in Medicine111: 101996. 10.1016/j.artmed.2020.101996. [DOI] [PubMed] [Google Scholar]
- *Chung, M., Lee M., Hong J., et al. 2020. “Pose‐Aware Instance Segmentation Framework From Cone Beam CT Images for Tooth Segmentation.” Computers in Biology and Medicine120: 103720. 10.1016/j.compbiomed.2020.103720. [DOI] [PubMed] [Google Scholar]
- *Cieślińska, K., Zaborowicz K., Zaborowicz M., and Biedziak B.. 2022. “Evaluation of the Second Premolar's Bud Position Using Computer Image Analysis and Neural Modelling Methods.” International Journal of Environmental Research and Public Health19, no. 22: 15240. 10.3390/ijerph192215240. [DOI] [PMC free article] [PubMed] [Google Scholar]
- *Cui, Z., Fang Y., Mei L., et al. 2022. “A Fully Automatic AI System for Tooth and Alveolar Bone Segmentation From Cone‐Beam CT Images.” Nature Communications13, no. 1: 2096. 10.1038/s41467-022-29637-2. [DOI] [PMC free article] [PubMed] [Google Scholar]
- *Cui, Z., Li C., Chen N., et al. 2021. “TSegNet: An Efficient and Accurate Tooth Segmentation Network on 3D Dental Model.” Medical Image Analysis69: 101949. 10.1016/j.media.2020.101949. [DOI] [PubMed] [Google Scholar]
- *Danks, R. P., Bano S., Orishko A., et al. 2021. “Automating Periodontal Bone Loss Measurement via Dental Landmark Localisation.” International Journal of Computer Assisted Radiology and Surgery16, no. 7: 1189–1199. 10.1007/s11548-021-02431-z. [DOI] [PMC free article] [PubMed] [Google Scholar]
- *Dayı, B., Üzen H., Çiçek İ. B., and Duman Ş. B.. 2023. “A Novel Deep Learning‐Based Approach for Segmentation of Different Type Caries Lesions on Panoramic Radiographs.” Diagnostics13, no. 2: 202. 10.3390/diagnostics13020202. [DOI] [PMC free article] [PubMed] [Google Scholar]
- Ding, H., Wu J., Zhao W., Matinlinna J. P., Burrow M. F., and Tsoi J. K. H.. 2023. “Artificial Intelligence in Dentistry—A Review.” Frontiers in Dental Medicine4: 1085251. 10.3389/fdmed.2023.1085251. [DOI] [Google Scholar]
- *Ding, M., Kang Y., Yuan Z., Shan X., and Cai Z.. 2021. “Detection of Facial Landmarks by a Convolutional Neural Network in Patients With Oral and Maxillofacial Disease.” International Journal of Oral and Maxillofacial Surgery50, no. 11: 1443–1449. 10.1016/j.ijom.2021.01.002. [DOI] [PubMed] [Google Scholar]
- Dong, F., Tao C., Wu J., et al. 2018. Detection of Cervical Lymph Node Metastasis From Oral Cavity Cancer Using a Non‐Radiating, Noninvasive Digital Infrared Thermal Imaging System (Vol. 8). 10.1038/s41598-018-24195-4. [DOI] [PMC free article] [PubMed]
- *Dot, G., Schouman T., Chang S., et al. 2022. “Automatic 3‐Dimensional Cephalometric Landmarking via Deep Learning.” Journal of Dental Research101, no. 11: 1380–1387. 10.1177/00220345221112333. [DOI] [PubMed] [Google Scholar]
- Du, X., Chen Y., Zhao J., and Xi Y.. 2018. “A Convolutional Neural Network Based Auto‐Positioning Method for Dental Arch in Rotational Panoramic Radiography.” Annual International Conference of the IEEE Engineering in Medicine and Biology Society. IEEE Engineering in Medicine and Biology Society. Annual International Conference, 2018, 2615–2618. 10.1109/EMBC.2018.8512732. [DOI] [PubMed]
- *Dubuc, A., Zitouni A., Thomas C., et al. 2022. “Improvement of Mucosal Lesion Diagnosis With Machine Learning Based on Medical and Semiological Data: An Observational Study.” Journal of Clinical Medicine11, no. 21: 6596. 10.3390/jcm11216596. [DOI] [PMC free article] [PubMed] [Google Scholar]
- *Duman, S., Yılmaz E. F., Eşer G., et al. 2023. “Detecting the Presence of Taurodont Teeth on Panoramic Radiographs Using a Deep Learning‐Based Convolutional Neural Network Algorithm.” Oral Radiology39, no. 1: 207–214. 10.1007/s11282-022-00622-1. [DOI] [PubMed] [Google Scholar]
- *Edvardsen, I. P., Teterina A., Johansen T., Myhre J. N., Godtliebsen F., and Bolstad N. L.. 2022. “Automatic Detection of the Mental Foramen for Estimating Mandibular Cortical Width in Dental Panoramic Radiographs: The Seventh Survey of the Tromsø Study (Tromsø7) in 2015–2016.” Journal of International Medical Research50, no. 11. 10.1177/03000605221135147. [DOI] [PMC free article] [PubMed] [Google Scholar]
- *Endres, M. G., Hillen F., Salloumis M., et al. 2020. “Development of a Deep Learning Algorithm for Periapical Disease Detection in Dental Radiographs.” Diagnostics10, no. 6: 430. 10.3390/diagnostics10060430. [DOI] [PMC free article] [PubMed] [Google Scholar]
- *Estai, M., Tennant M., Gebauer D., et al. 2022. “Evaluation of a Deep Learning System for Automatic Detection of Proximal Surface Dental Caries on Bitewing Radiographs.” Oral Surgery, Oral Medicine, Oral Pathology and Oral Radiology134, no. 2: 262–270. 10.1016/j.oooo.2022.03.008. [DOI] [PubMed] [Google Scholar]
- *Etemad, L., Wu T.‐H., Heiner P., et al. 2021. “Machine Learning From Clinical Data Sets of a Contemporary Decision for Orthodontic Tooth Extraction.” Orthodontics & Craniofacial Research24: 193–200. 10.1111/ocr.12502. [DOI] [PubMed] [Google Scholar]
- *Ezhov, M., Gusarev M., Golitsyna M., et al. 2021. “Clinically Applicable Artificial Intelligence System for Dental Diagnosis With CBCT.” Scientific Reports11, no. 1: 15006. 10.1038/s41598-021-94093-9. [DOI] [PMC free article] [PubMed] [Google Scholar]
- *Figueroa, K. C., Song B., Sunny S., et al. 2022. “Interpretable Deep Learning Approach for Oral Cancer Classification Using Guided Attention Inference Network.” Journal of Biomedical Optics27, no. 1: 015001. 10.1117/1.JBO.27.1.015001. [DOI] [PMC free article] [PubMed] [Google Scholar]
- *Firestone, A. R., Sema D., Heaven T. J., and Weems R. A.. 1998. “The Effect of a Knowledge‐Based, Image Analysis and Clinical Decision Support System on Observer Performance in the Diagnosis of Approximal Caries From Radiographic Images.” Caries Research32, no. 2: 127–134. 10.1159/000016442. [DOI] [PubMed] [Google Scholar]
- *Flügge, T., Gaudin R., Sabatakakis A., et al. 2023. “Detection of Oral Squamous Cell Carcinoma in Clinical Photographs Using a Vision Transformer.” Scientific Reports13, no. 1: 2296. 10.1038/s41598-023-29204-9. [DOI] [PMC free article] [PubMed] [Google Scholar]
- Fu, Q., Chen Y., Li Z., et al. 2020. “A Deep Learning Algorithm for Detection of Oral Cavity Squamous Cell Carcinoma From Photographic Images: A Retrospective Study.” EClinicalMedicine27: 100558. 10.1016/j.eclinm.2020.100558. [DOI] [PMC free article] [PubMed] [Google Scholar]
- *Fujima, N., Andreu‐Arasa V. C., Meibom S. K., et al. 2020. “Deep Learning Analysis Using FDG‐PET to Predict Treatment Outcome in Patients With Oral Cavity Squamous Cell Carcinoma.” European Radiology30, no. 11: 6322–6330. 10.1007/s00330-020-06982-8. [DOI] [PubMed] [Google Scholar]
- *Fujima, N., Andreu‐Arasa V. C., Meibom S. K., et al. 2021. “Prediction of the Treatment Outcome Using Machine Learning With FDG‐PET Image‐Based Multiparametric Approach in Patients With Oral Cavity Squamous Cell Carcinoma.” Clinical Radiology76, no. 9: 711.e1–711.e7. 10.1016/j.crad.2021.03.017. [DOI] [PubMed] [Google Scholar]
- *Fukuda, M., Inamoto K., Shibata N., et al. 2020. “Evaluation of an Artificial Intelligence System for Detecting Vertical Root Fracture on Panoramic Radiography.” Oral Radiology36, no. 4: 337–343. 10.1007/s11282-019-00409-x. [DOI] [PubMed] [Google Scholar]
- *Galibourg, A., Cussat‐Blanc S., Dumoncel J., Telmon N., Monsarrat P., and Maret D.. 2021. “Comparison of Different Machine Learning Approaches to Predict Dental Age Using Demirjian's Staging Approach.” International Journal of Legal Medicine135, no. 2: 665–675. 10.1007/s00414-020-02489-5. [DOI] [PubMed] [Google Scholar]
- *Gao, S., Li X., Li X., Li Z., and Deng Y.. 2022. “Transformer Based Tooth Classification From Cone‐Beam Computed Tomography for Dental Charting.” Computers in Biology and Medicine148: 105880. 10.1016/j.compbiomed.2022.105880. [DOI] [PubMed] [Google Scholar]
- García‐Cañas, Á., Bonfanti‐Gris M., Paraíso‐Medina S., Martínez‐Rus F., and Pradíes G.. 2022. “Diagnosis of Interproximal Caries Lesions in Bitewing Radiographs Using a Deep Convolutional Neural Network‐Based Software.” Caries Research56, no. 5: 503–511. 10.1159/000527491. [DOI] [PubMed] [Google Scholar]
- *Geetha, V., Aprameya K. S., and Hinduja D. M.. 2020. “Dental Caries Diagnosis in Digital Radiographs Using Back‐Propagation Neural Network.” Health Information Science and Systems8, no. 1: 8. 10.1007/s13755-019-0096-y. [DOI] [PMC free article] [PubMed] [Google Scholar]
- *Gerhardt, M. N., Fontenele R. C., Leite A. F., et al. 2022. “Automated Detection and Labelling of Teeth and Small Edentulous Regions on Cone‐Beam Computed Tomography Using Convolutional Neural Networks.” Journal of Dentistry122: 104139. 10.1016/j.jdent.2022.104139. [DOI] [PubMed] [Google Scholar]
- *Görürgöz, C., Orhan K., Bayrakdar I. S., et al. 2022. “Performance of a Convolutional Neural Network Algorithm for Tooth Detection and Numbering on Periapical Radiographs.” Dentomaxillofacial Radiology51, no. 3: 20210246. 10.1259/dmfr.20210246. [DOI] [PMC free article] [PubMed] [Google Scholar]
- *Guo, J., Wu Y., Chen L., Ge G., Tang Y., and Wang W.. 2022. “Automatic Detection of Cracks in Cracked Tooth Based on Binary Classification Convolutional Neural Networks.” Applied Bionics and Biomechanics2022: 1–12. 10.1155/2022/9333406. [DOI] [PMC free article] [PubMed] [Google Scholar]
- *Hadj Saïd, M., Le Roux M.‐K., Catherine J.‐H., and Lan R.. 2020. “Development of an Artificial Intelligence Model to Identify a Dental Implant From a Radiograph.” International Journal of Oral & Maxillofacial Implants35, no. 6: 1077–1082. 10.11607/jomi.8060. [DOI] [PubMed] [Google Scholar]
- *Hamdan, M. H., Tuzova L., Mol A., Tawil P. Z., Tuzoff D., and Tyndall D. A.. 2022. “The Effect of a Deep‐Learning Tool on Dentists’ Performances in Detecting Apical Radiolucencies on Periapical Radiographs.” Dentomaxillofacial Radiology51, no. 7: 20220122. 10.1259/dmfr.20220122. [DOI] [PMC free article] [PubMed] [Google Scholar]
- *Han, M., Du S., Ge Y., et al. 2022. “With or Without Human Interference for Precise Age Estimation Based on Machine Learning?” International Journal of Legal Medicine136, no. 3: 821–831. 10.1007/s00414-022-02796-z. [DOI] [PubMed] [Google Scholar]
- *Hao, J., Liao W., Zhang Y. L., et al. 2022. “Toward Clinically Applicable 3‐Dimensional Tooth Segmentation via Deep Learning.” Journal of Dental Research101, no. 3: 304–311. 10.1177/00220345211040459. [DOI] [PubMed] [Google Scholar]
- Hernandez, S., Sjogreen C., Gay S. S., et al. 2021. “Development and Dosimetric Assessment of an Automatic Dental Artifact Classification Tool to Guide Artifact Management Techniques in a Fully Automated Treatment Planning Workflow.” Computerized Medical Imaging and Graphics90: 101907. 10.1016/j.compmedimag.2021.101907. [DOI] [PMC free article] [PubMed] [Google Scholar]
- *Hiraiwa, T., Ariji Y., Fukuda M., et al. 2019. “A Deep‐Learning Artificial Intelligence System for Assessment of Root Morphology of the Mandibular First Molar on Panoramic Radiography.” Dentomaxillofacial Radiology48, no. 3: 20180218. 10.1259/dmfr.20180218. [DOI] [PMC free article] [PubMed] [Google Scholar]
- *Ter Horst, R., van Weert H., Loonen T., et al. 2021. “Three‐Dimensional Virtual Planning in Mandibular Advancement Surgery: Soft Tissue Prediction Based on Deep Learning.” Journal of Cranio‐Maxillofacial Surgery49, no. 9: 775–782. 10.1016/j.jcms.2021.04.001. [DOI] [PubMed] [Google Scholar]
- *Hosntalab, M., Aghaeizadeh Zoroofi R., Abbaspour tehrani‐Fard A., and Shirani G.. 2010. “Classification and Numbering of Teeth in Multi‐Slice CT Images Using Wavelet‐Fourier Descriptor.” International Journal of Computer Assisted Radiology and Surgery5, no. 3: 237–249. 10.1007/s11548-009-0389-8. [DOI] [PubMed] [Google Scholar]
- *Hou, S., Zhou T., Liu Y., Dang P., Lu H., and Shi H.. 2023. “Teeth U‐Net: A Segmentation Model of Dental Panoramic X‐Ray Images for Context Semantics and Contrast Enhancement.” Computers in Biology and Medicine152: 106296. 10.1016/j.compbiomed.2022.106296. [DOI] [PubMed] [Google Scholar]
- *Hu, Z., Cao D., Hu Y., et al. 2022. “Diagnosis of In Vivo Vertical Root Fracture Using Deep Learning on Cone‐Beam CT Images.” BMC Oral Health22, no. 1: 382. 10.1186/s12903-022-02422-9. [DOI] [PMC free article] [PubMed] [Google Scholar]
- Huang, N., Liu P., Yan Y., et al. 2022. “Predicting the Risk of Dental Implant Loss Using Deep Learning.” Journal of Clinical Periodontology49, no. 9: 872–883. 10.1111/jcpe.13689. [DOI] [PubMed] [Google Scholar]
- Huang, Z., Xia T., Kim J., Zhang L., and Li B.. 2021. Combining CNN With Pathological Information for the Detection of Transmissive Lesions of Jawbones From CBCT Images. Institute of Electrical and Electronics Engineers Inc. 10.1109/EMBC46164.2021.9630692. [DOI] [PubMed] [Google Scholar]
- *Hur, S.‐H., Lee E.‐Y., Kim M.‐K., Kim S., Kang J.‐Y., and Lim J. S.. 2021. “Machine Learning to Predict Distal Caries in Mandibular Second Molars Associated With Impacted Third Molars.” Scientific Reports11, no. 1: 15447. 10.1038/s41598-021-95024-4. [DOI] [PMC free article] [PubMed] [Google Scholar]
- *Hwang, J. J., Lee J.‐H., Han S.‐S., et al. 2017. “Strut Analysis for Osteoporosis Detection Model Using Dental Panoramic Radiography.” Dentomaxillofacial Radiology46, no. 7: 20170006. 10.1259/dmfr.20170006. [DOI] [PMC free article] [PubMed] [Google Scholar]
- *Ishibashi, K., Ariji Y., Kuwada C., et al. 2022. “Efficacy of a Deep Leaning Model Created With the Transfer Learning Method in Detecting Sialoliths of the Submandibular Gland on Panoramic Radiography.” Oral Surgery, Oral Medicine, Oral Pathology and Oral Radiology133, no. 2: 238–244. 10.1016/j.oooo.2021.08.010. [DOI] [PubMed] [Google Scholar]
- *Issa, J., Jaber M., Rifai I., Mozdziak P., Kempisty B., and Dyszkiewicz‐Konwińska M.. 2023. “Diagnostic Test Accuracy of Artificial Intelligence in Detecting Periapical Periodontitis on Two‐Dimensional Radiographs: A Retrospective Study and Literature Review.” Medicina (Kaunas, Lithuania)59, no. 4: 768. 10.3390/medicina59040768. [DOI] [PMC free article] [PubMed] [Google Scholar]
- *Issa, N., Leonas J., Jham B. C., Mitchell J. C., and Cuevas‐Nunez M. C.. 2022. “Early Detection of Oral Potentially Malignant Disorders Using Machine Learning: A Retrospective Pilot Study.” General Dentistry70, no. 6: 60–64. [PubMed] [Google Scholar]
- *James, B. L., Sunny S. P., Heidari A. E., et al. 2021. “Validation of a Point‐of‐Care Optical Coherence Tomography Device With Machine Learning Algorithm for Detection of Oral Potentially Malignant and Malignant Lesions.” Cancers13, no. 14: 3583. 10.3390/cancers13143583. [DOI] [PMC free article] [PubMed] [Google Scholar]
- *Jang, W. S., Kim S., Yun P. S., et al. 2022. “Accurate Detection for Dental Implant and Peri‐Implant Tissue by Transfer Learning of Faster R‐Cnn: A Diagnostic Accuracy Study.” BMC Oral Health22, no. 1: 591. 10.1186/s12903-022-02539-x. [DOI] [PMC free article] [PubMed] [Google Scholar]
- Janiesch, C., Zschech P., and Heinrich K.. 2021. “Machine Learning and Deep Learning.” Electronic Markets31, no. 3: 685–695. 10.1007/s12525-021-00475-2. [DOI] [Google Scholar]
- *Jeon, S., and Lee K. C.. 2021. “Comparison of Cephalometric Measurements Between Conventional and Automatic Cephalometric Analysis Using Convolutional Neural Network.” Progress in Orthodontics22, no. 1: 14. 10.1186/s40510-021-00358-4. [DOI] [PMC free article] [PubMed] [Google Scholar]
- *Jeon, S.‐J., Yun J.‐P., Yeom H.‐G., et al. 2021. “Deep‐Learning for Predicting C‐Shaped Canals in Mandibular Second Molars on Panoramic Radiographs.” Dentomaxillofacial Radiology50, no. 5: 20200513. 10.1259/dmfr.20200513. [DOI] [PMC free article] [PubMed] [Google Scholar]
- *Jeong, S. H., Woo M. W., Shin D. S., et al. 2022. “Three‐Dimensional Postoperative Results Prediction for Orthognathic Surgery Through Deep Learning‐Based Alignment Network.” Journal of Personalized Medicine12, no. 6: 998. 10.3390/jpm12060998. [DOI] [PMC free article] [PubMed] [Google Scholar]
- *Jeong, S. H., Yun J. P., Yeom H.‐G., Lim H. J., Lee J., and Kim B. C.. 2020. “Deep Learning Based Discrimination of Soft Tissue Profiles Requiring Orthognathic Surgery by Facial Photographs.” Scientific Reports10, no. 1: 16235. 10.1038/s41598-020-73287-7. [DOI] [PMC free article] [PubMed] [Google Scholar]
- *Jiang, F., Guo Y., Yang C., et al. 2023. “Artificial Intelligence System for Automated Landmark Localization and Analysis of Cephalometry.” Dentomaxillofacial Radiology52, no. 1: 20220081. 10.1259/dmfr.20220081. [DOI] [PMC free article] [PubMed] [Google Scholar]
- *Jiang, H., Zhang P., Che C., and Jin B.. 2021. “RDFNet: A Fast Caries Detection Method Incorporating Transformer Mechanism.” Computational and Mathematical Methods in Medicine2021: 1–9. 10.1155/2021/9773917. [DOI] [PMC free article] [PubMed] [Google Scholar]
- *Jiang, L., Chen D., Cao Z., Wu F., Zhu H., and Zhu F.. 2022. “A Two‐Stage Deep Learning Architecture for Radiographic Staging of Periodontal Bone Loss.” BMC Oral Health22, no. 1: 106. 10.1186/s12903-022-02119-z. [DOI] [PMC free article] [PubMed] [Google Scholar]
- Jingarwar, M., Bajwa N., and Pathak A.. 2014. “Minimal Intervention Dentistry – A New Frontier in Clinical Dentistry.” Journal of Clinical Diagnostic Research8, no. 7: Ze04–Ze08. 10.7860/jcdr/2014/9128.4583. [DOI] [PMC free article] [PubMed] [Google Scholar]
- *Jones, K. A., Jones N., Tenuta L. M. A., et al. 2022. “Convolution Neural Networks and Targeted Fluorescent Nanoparticles to Detect and ICDAS Score Caries.” Caries Research56, no. 4: 419–428. 10.1159/000527118. [DOI] [PubMed] [Google Scholar]
- *Joo, S., Jung W., and Oh S. E.. 2023. “Variational Autoencoder‐Based Estimation of Chronological Age and Changes in Morphological Features of Teeth.” Scientific Reports13, no. 1: 704. 10.1038/s41598-023-27950-4. [DOI] [PMC free article] [PubMed] [Google Scholar]
- *Jubair, F., Al‐karadsheh O., Malamos D., Al Mahdi S., Saad Y., and Hassona Y.. 2022. “A Novel Lightweight Deep Convolutional Neural Network for Early Detection of Oral Cancer.” Oral Diseases28, no. 4: 1123–1130. 10.1111/odi.13825. [DOI] [PubMed] [Google Scholar]
- *Jung, W., Lee K.‐E., Suh B.‐J., Seok H., and Lee D.‐W.. 2023. “Deep Learning for Osteoarthritis Classification in Temporomandibular Joint.” Oral Diseases29, no. 3: 1050–1059. 10.1111/odi.14056. [DOI] [PubMed] [Google Scholar]
- *Jurczyszyn, K., Gedrange T., and Kozakiewicz M.. 2020. “Theoretical Background to Automated Diagnosing of Oral Leukoplakia: A Preliminary Report.” Journal of Healthcare Engineering2020: 1–7. 10.1155/2020/8831161. [DOI] [PMC free article] [PubMed] [Google Scholar]
- *Kabir, T., Lee C.‐T., Chen L., Jiang X., and Shams S.. 2022. “A Comprehensive Artificial Intelligence Framework for Dental Diagnosis and Charting.” BMC Oral Health22, no. 1: 480. 10.1186/s12903-022-02514-6. [DOI] [PMC free article] [PubMed] [Google Scholar]
- *Kats, L., Vered M., Kharouba J., and Blumer S.. 2021. “Transfer Deep Learning for Dental and Maxillofacial Imaging Modality Classification: A Preliminary Study.” Journal of Clinical Pediatric Dentistry45, no. 4: 233–238. 10.17796/1053-4625-45.4.3. [DOI] [PubMed] [Google Scholar]
- *Kavitha, M. S., An S. Y., An C. H., et al. 2015. “Texture Analysis of Mandibular Cortical Bone on Digital Dental Panoramic Radiographs for the Diagnosis of Osteoporosis in Korean Women.” Oral Surgery, Oral Medicine, Oral Pathology and Oral Radiology119, no. 3: 346–356. 10.1016/j.oooo.2014.11.009. [DOI] [PubMed] [Google Scholar]
- *Kavitha, M. S., An S. Y., An C. H., et al. 2015. “Texture Analysis of Mandibular Cortical Bone on Digital Dental Panoramic Radiographs for the Diagnosis of Osteoporosis in Korean Women.” Oral Surgery, Oral Medicine, Oral Pathology and Oral Radiology119, no. 3: 346–356. 10.1016/j.oooo.2014.11.009. [DOI] [PubMed] [Google Scholar]
- *Kavitha, M. S., Asano A., Taguchi A., Kurita T., and Sanada M.. 2012. “Diagnosis of Osteoporosis From Dental Panoramic Radiographs Using the Support Vector Machine Method in a Computer‐Aided System.” BMC Medical Imaging12: 1. 10.1186/1471-2342-12-1. [DOI] [PMC free article] [PubMed] [Google Scholar]
- Kearney, V. P., Yansane A.‐I. M., Brandon R. G., et al. 2022. “A Generative Adversarial Inpainting Network to Enhance Prediction of Periodontal Clinical Attachment Level.” Journal of Dentistry123: 104211. 10.1016/j.jdent.2022.104211. [DOI] [PubMed] [Google Scholar]
- *Kempers, S., van Lierop P., Hsu T.‐M. H., et al. 2023. “Positional Assessment of Lower Third Molar and Mandibular Canal Using Explainable Artificial Intelligence.” Journal of Dentistry133: 104519. 10.1016/j.jdent.2023.104519. [DOI] [PubMed] [Google Scholar]
- *Kim, D. W., Kim J., Kim T., et al. 2021. “Prediction of Hand‐Wrist Maturation Stages Based on Cervical Vertebrae Images Using Artificial Intelligence.” Orthodontics & Craniofacial Research24, no. S2: 68–75. 10.1111/ocr.12514. [DOI] [PubMed] [Google Scholar]
- *Kim, H., Kim C.‐S., Lee J.‐M., et al. 2023. “Prediction of Fishman's Skeletal Maturity Indicators Using Artificial Intelligence.” Scientific Reports13, no. 1: 5870. 10.1038/s41598-023-33058-6. [DOI] [PMC free article] [PubMed] [Google Scholar]
- *Kim, J., Hwang J. J., Jeong T., Cho B.‐H., and Shin J.. 2022. “Deep Learning‐Based Identification of Mesiodens Using Automatic Maxillary Anterior Region Estimation in Panoramic Radiography of Children.” Dentomaxillofacial Radiology51, no. 7: 20210528. 10.1259/dmfr.20210528. [DOI] [PMC free article] [PubMed] [Google Scholar]
- *Kim, J., Kim I., Kim Y. J., et al. 2021. “Accuracy of Automated Identification of Lateral Cephalometric Landmarks Using Cascade Convolutional Neural Networks on Lateral Cephalograms From Nationwide Multi‐Centres.” Orthodontics & Craniofacial Research24, no. S2: 59–67. 10.1111/ocr.12493. [DOI] [PubMed] [Google Scholar]
- *Kim, J.‐E., Nam N.‐E., Shim J.‐S., Jung Y.‐H., Cho B.‐H., and Hwang J. J.. 2020. “Transfer Learning via Deep Neural Networks for Implant Fixture System Classification Using Periapical Radiographs.” Journal of Clinical Medicine9, no. 4: 1117. 10.3390/jcm9041117. [DOI] [PMC free article] [PubMed] [Google Scholar]
- *Kim, S., Lee Y.‐H., Noh Y.‐K., Park F. C., and Auh Q.‐S.. 2021. “Age‐Group Determination of Living Individuals Using First Molar Images Based on Artificial Intelligence.” Scientific Reports11, no. 1: 1073. 10.1038/s41598-020-80182-8. [DOI] [PMC free article] [PubMed] [Google Scholar]
- *Kim, Y. H., Shin J. Y., Lee A., Park S., Han S.‐S., and Hwang H. J.. 2021. “Automated Cortical Thickness Measurement of the Mandibular Condyle Head on CBCT Images Using a Deep Learning Method.” Scientific Reports11, no. 1: 14852. 10.1038/s41598-021-94362-7. [DOI] [PMC free article] [PubMed] [Google Scholar]
- *Kise, Y., Ikeda H., Fujii T., et al. 2019. “Preliminary Study on the Application of Deep Learning System to Diagnosis of Sjögren's Syndrome on Ct Images.” Dento Maxillo Facial Radiology48, no. 6: 20190019. 10.1259/dmfr.20190019. [DOI] [PMC free article] [PubMed] [Google Scholar]
- *Kök, H., Izgi M. S., and Acilar A. M.. 2021. “Determination of Growth and Development Periods in Orthodontics With Artificial Neural Network.” Orthodontics & Craniofacial Research24, no. S2: 76–83. 10.1111/ocr.12443. [DOI] [PubMed] [Google Scholar]
- *Kositbowornchai, S., Plermkamon S., and Tangkosol T.. 2013. “Performance of an Artificial Neural Network for Vertical Root Fracture Detection: An Ex Vivo Study.” Dental Traumatology29, no. 2: 151–155. 10.1111/j.1600-9657.2012.01148.x. [DOI] [PubMed] [Google Scholar]
- *Krois, J., Ekert T., Meinhold L., et al. 2019. “Deep Learning for the Radiographic Detection of Periodontal Bone Loss.” Scientific Reports9, no. 1: 8495. 10.1038/s41598-019-44839-3. [DOI] [PMC free article] [PubMed] [Google Scholar]
- *Krois, J., Schneider L., and Schwendicke F.. 2021. “Impact of Image Context on Deep Learning for Classification of Teeth on Radiographs.” Journal of Clinical Medicine10, no. 8: 1635. 10.3390/jcm10081635. [DOI] [PMC free article] [PubMed] [Google Scholar]
- *Kubo, K., Kawahara D., Murakami Y., et al. 2022. “Development of a Radiomics and Machine Learning Model for Predicting Occult Cervical Lymph Node Metastasis in Patients With Tongue Cancer.” Oral Surgery, Oral Medicine, Oral Pathology and Oral Radiology134, no. 1: 93–101. 10.1016/j.oooo.2021.12.122. [DOI] [PubMed] [Google Scholar]
- *Kühnisch, J., Meyer O., Hesenius M., Hickel R., and Gruhn V.. 2022. “Caries Detection on Intraoral Images Using Artificial Intelligence.” Journal of Dental Research101, no. 2: 158–165. 10.1177/00220345211032524. [DOI] [PMC free article] [PubMed] [Google Scholar]
- *Kunz, F., Stellzig‐Eisenhauer A., Zeman F., and Boldt J.. 2020. “Artificial Intelligence in Orthodontics: Evaluation of a Fully Automated Cephalometric Analysis Using a Customized Convolutional Neural Network.” Journal of Orofacial Orthopedics/Fortschritte der Kieferorthopädie81, no. 1: 52–68. 10.1007/s00056-019-00203-8. [DOI] [PubMed] [Google Scholar]
- *Kuwada, C., Ariji Y., Fukuda M., et al. 2020. “Deep Learning Systems for Detecting and Classifying the Presence of Impacted Supernumerary Teeth in the Maxillary Incisor Region on Panoramic Radiographs.” Oral Surgery, Oral Medicine, Oral Pathology and Oral Radiology130, no. 4: 464–469. 10.1016/j.oooo.2020.04.813. [DOI] [PubMed] [Google Scholar]
- *Kuwada, C., Ariji Y., Kise Y., et al. 2021. “Detection and Classification of Unilateral Cleft Alveolus With and Without Cleft Palate on Panoramic Radiographs Using a Deep Learning System.” Scientific Reports11, no. 1: 16044. 10.1038/s41598-021-95653-9. [DOI] [PMC free article] [PubMed] [Google Scholar]
- *Kwak, G. H., Kwak E.‐J., Song J. M., et al. 2020. “Automatic Mandibular Canal Detection Using a Deep Convolutional Neural Network.” Scientific Reports10, no. 1: 5711. 10.1038/s41598-020-62586-8. [DOI] [PMC free article] [PubMed] [Google Scholar]
- *Kwon, D., Ahn J., Kim C.‐S., Kang D., and Paeng J.‐Y.. 2022. “A Deep Learning Model Based on Concatenation Approach to Predict the Time to Extract a Mandibular Third Molar Tooth.” BMC Oral Health22, no. 1: 571. 10.1186/s12903-022-02614-3. [DOI] [PMC free article] [PubMed] [Google Scholar]
- *Kyventidis, N., and Angelopoulos C.. 2021. “Intraoral Radiograph Anatomical Region Classification Using Neural Networks.” International Journal of Computer Assisted Radiology and Surgery16, no. 3: 447–455. 10.1007/s11548-021-02321-4. [DOI] [PubMed] [Google Scholar]
- *Le, V. N. T., Kang J., Oh I.‐S., Kim J.‐G., Yang Y.‐M., and Lee D.‐W.. 2022. “Effectiveness of Human–Artificial Intelligence Collaboration in Cephalometric Landmark Detection.” Journal of Personalized Medicine12, no. 3: 387. 10.3390/jpm12030387. [DOI] [PMC free article] [PubMed] [Google Scholar]
- *Lee, A., Kim M. S., Han S.‐S., Park P., Lee C., and Yun J. P.. 2021. “Deep Learning Neural Networks to Differentiate Stafne's Bone Cavity From Pathological Radiolucent Lesions of the Mandible in Heterogeneous Panoramic Radiography.” PLoS One16, no. 7: e0254997. 10.1371/journal.pone.0254997. [DOI] [PMC free article] [PubMed] [Google Scholar]
- *Lee, C.‐T., Kabir T., Nelson J., et al. 2022. “Use of the Deep Learning Approach to Measure Alveolar Bone Level.” Journal of Clinical Periodontology49, no. 3: 260–269. 10.1111/jcpe.13574. [DOI] [PMC free article] [PubMed] [Google Scholar]
- *Lee, J. H., and Jeong S. N.. 2020. “Efficacy of Deep Convolutional Neural Network Algorithm for the Identification and Classification of Dental Implant Systems, Using Panoramic and Periapical Radiographs: A Pilot Study.” Medicine99, no. 26: e20787. 10.1097/md.0000000000020787. [DOI] [PMC free article] [PubMed] [Google Scholar]
- *Lee, J.‐H., Kim D.‐H., and Jeong S.‐N.. 2020. “Diagnosis of Cystic Lesions Using Panoramic and Cone Beam Computed Tomographic Images Based on Deep Learning Neural Network.” Oral Diseases26, no. 1: 152–158. 10.1111/odi.13223. [DOI] [PubMed] [Google Scholar]
- *Lee, J. H., Kim D. H., Jeong S. N., and Choi S. H.. 2018. “Detection and Diagnosis of Dental Caries Using a Deep Learning‐Based Convolutional Neural Network Algorithm.” Journal of Dentistry77: 106–111. 10.1016/j.jdent.2018.07.015. [DOI] [PubMed] [Google Scholar]
- *Lee, J.‐H., Yu H.‐J., Kim M.‐J., Kim J.‐W., and Choi J.. 2020a. “Automated Cephalometric Landmark Detection With Confidence Regions Using Bayesian Convolutional Neural Networks.” BMC Oral Health20, no. 1. 10.1186/s12903-020-01256-7. [DOI] [PMC free article] [PubMed] [Google Scholar]
- *Lee, K.‐S., Jung S.‐K., Ryu J.‐J., Shin S.‐W., and Choi J.. 2020. “Evaluation of Transfer Learning With Deep Convolutional Neural Networks for Screening Osteoporosis in Dental Panoramic Radiographs.” Journal of Clinical Medicine9, no. 2: 392. 10.3390/jcm9020392. [DOI] [PMC free article] [PubMed] [Google Scholar]
- *Lee, S.‐C., Hwang H.‐S., and Lee K. C.. 2022. “Accuracy of Deep Learning‐Based Integrated Tooth Models by Merging Intraoral Scans and CBCT Scans for 3D Evaluation of Root Position During Orthodontic Treatment.” Progress in Orthodontics23, no. 1: 15. 10.1186/s40510-022-00410-x. [DOI] [PMC free article] [PubMed] [Google Scholar]
- *Lee, Y. H., Won J. H., Kim S., Auh Q. S., and Noh Y. K.. 2022. “Advantages of Deep Learning With Convolutional Neural Network in Detecting Disc Displacement of the Temporomandibular Joint in Magnetic Resonance Imaging.” Scientific Reports12, no. 1: 11352. 10.1038/s41598-022-15231-5. [DOI] [PMC free article] [PubMed] [Google Scholar]
- *Li, C.‐W., Lin S.‐Y., Chou H.‐S., et al. 2021. “Detection of Dental Apical Lesions Using CNNs on Periapical Radiograph.” Sensors21, no. 21: 7049. 10.3390/s21217049. [DOI] [PMC free article] [PubMed] [Google Scholar]
- *Li, H., Zhou J., Zhou Y., et al. 2021. “An Interpretable Computer‐Aided Diagnosis Method for Periodontitis From Panoramic Radiographs.” Frontiers in Physiology12: 655556. 10.3389/fphys.2021.655556. [DOI] [PMC free article] [PubMed] [Google Scholar]
- *Li, Q., Chen K., Han L., Zhuang Y., Li J., and Lin J.. 2020. “Automatic Tooth Roots Segmentation of Cone Beam Computed Tomography Image Sequences Using U‐Net and RNN.” Journal of X‐ray Science and Technology28, no. 5: 905–922. 10.3233/XST-200678. [DOI] [PubMed] [Google Scholar]
- *Li, S., Liu J., Zhou Z., et al. 2022. “Artificial Intelligence for Caries and Periapical Periodontitis Detection.” Journal of Dentistry122: 104107. 10.1016/j.jdent.2022.104107. [DOI] [PubMed] [Google Scholar]
- *Li, W., Liang Y., Zhang X., et al. 2021. “A Deep Learning Approach to Automatic Gingivitis Screening Based on Classification and Localization in RGB Photos.” Scientific Reports11, no. 1: 16831. 10.1038/s41598-021-96091-3. [DOI] [PMC free article] [PubMed] [Google Scholar]
- *Li, Y., Jin H., and Li Z.. 2022. “A Weakly Supervised Learning‐Based Segmentation Network for Dental Diseases.” Mathematical Biosciences and Engineering20, no. 2: 2039–2060. 10.3934/mbe.2023094. [DOI] [PubMed] [Google Scholar]
- *Li, Y., Zeng G., Zhang Y., et al. 2022. “AGMB‐Transformer: Anatomy‐Guided Multi‐Branch Transformer Network for Automated Evaluation of Root Canal Therapy.” IEEE Journal of Biomedical and Health Informatics26, no. 4: 1684–1695. 10.1109/JBHI.2021.3129245. [DOI] [PubMed] [Google Scholar]
- *Lian, L., Zhu T., Zhu F., and Zhu H.. 2021. “Deep Learning for Caries Detection and Classification.” Diagnostics11, no. 9: 1672. 10.3390/DIAGNOSTICS11091672. [DOI] [PMC free article] [PubMed] [Google Scholar]
- *Lim, H.‐K., Jung S.‐K., Kim S.‐H., Cho Y., and Song I.‐S.. 2021. “Deep Semi‐Supervised Learning for Automatic Segmentation of Inferior Alveolar Nerve Using a Convolutional Neural Network.” BMC Oral Health21, no. 1: 630. 10.1186/s12903-021-01983-5. [DOI] [PMC free article] [PubMed] [Google Scholar]
- *Lin, B., Cheng M., Wang S., Li F., and Zhou Q.. 2022. “Automatic Detection of Anteriorly Displaced Temporomandibular Joint Discs on Magnetic Resonance Images Using a Deep Learning Algorithm.” Dento Maxillo Facial Radiology51, no. 3: 20210341. 10.1259/dmfr.20210341. [DOI] [PMC free article] [PubMed] [Google Scholar]
- *Lin, H. H., Chiang W. C., Yang C. T., Cheng C. T., Zhang T., and Lo L. J.. 2021. “On Construction of Transfer Learning for Facial Symmetry Assessment Before and After Orthognathic Surgery.” Computer Methods and Programs in Biomedicine200: 105928. 10.1016/j.cmpb.2021.105928. [DOI] [PubMed] [Google Scholar]
- *Lin, X., Hong D., Zhang D., Huang M., and Yu H.. 2022. “Detecting Proximal Caries on Periapical Radiographs Using Convolutional Neural Networks With Different Training Strategies on Small Datasets.” Diagnostics12, no. 5: 1047. 10.3390/diagnostics12051047. [DOI] [PMC free article] [PubMed] [Google Scholar]
- *Lin, Y., Shi M., Xiang D., et al. 2022. “Construction of an End‐to‐End Regression Neural Network for the Determination of a Quantitative Index Sagittal Root Inclination.” Journal of Periodontology93, no. 12: 1951–1960. 10.1002/JPER.21-0492. [DOI] [PubMed] [Google Scholar]
- *Lingam, A. S., Koppolu P., Akhter F., et al. 2022. “Future Trends of Artificial Intelligence in Dentistry.” Journal of Nature and Science of Medicine5, no. 3: 221–224. 10.4103/jnsm.jnsm_2_22. [DOI] [Google Scholar]
- *Liu, F., Gao L., Wan J., et al. 2023. “Recognition of Digital Dental X‐Ray Images Using a Convolutional Neural Network.” Journal of Digital Imaging36, no. 1: 73–79. 10.1007/s10278-022-00694-9. [DOI] [PMC free article] [PubMed] [Google Scholar]
- *Liu, J., Liu Y., Li S., Ying S., Zheng L., and Zhao Z.. 2022. “Artificial Intelligence‐Aided Detection of Ectopic Eruption of Maxillary First Molars Based on Panoramic Radiographs.” Journal of Dentistry125: 104239. 10.1016/j.jdent.2022.104239. [DOI] [PubMed] [Google Scholar]
- *Liu, J. L., Li S. H., Cai Y. M., et al. 2021. “Automated Radiographic Evaluation of Adenoid Hypertrophy Based on VGG‐Lite.” Journal of Dental Research100, no. 12: 1337–1343. 10.1177/00220345211009474. [DOI] [PubMed] [Google Scholar]
- *Liu, M., Wang S., Chen H., and Liu Y.. 2022. “A Pilot Study of a Deep Learning Approach to Detect Marginal Bone Loss Around Implants.” BMC Oral Health22, no. 1: 11. 10.1186/s12903-021-02035-8. [DOI] [PMC free article] [PubMed] [Google Scholar]
- *Liu, M.‐Q., Xu Z.‐N., Mao W.‐Y., et al. 2022. “Deep Learning‐Based Evaluation of the Relationship Between Mandibular Third Molar and Mandibular Canal on CBCT.” Clinical Oral Investigations26, no. 1: 981–991. 10.1007/s00784-021-04082-5. [DOI] [PubMed] [Google Scholar]
- *Liu, T., Ye Y., Liu C., et al. 2023. “Key‐Point Based Automated Diagnosis for Alveolar Dehiscence in Mandibular Incisors Using Convolutional Neural Network.” Biomedical Signal Processing and Control85: 105082. 10.1016/j.bspc.2023.105082. [DOI] [Google Scholar]
- *Liu, Z., Liu J., Zhou Z., et al. 2021. “Differential Diagnosis of Ameloblastoma and Odontogenic Keratocyst by Machine Learning of Panoramic Radiographs.” International Journal of Computer Assisted Radiology and Surgery16, no. 3: 415–422. 10.1007/s11548-021-02309-0. [DOI] [PMC free article] [PubMed] [Google Scholar]
- *Lu, G., Shu H., Bao H., et al. 2023. “CMF‐Net: Craniomaxillofacial Landmark Localization on CBCT Images Using Geometric Constraint and Transformer.” Physics in Medicine & Biology68, no. 9: 095020. 10.1088/1361-6560/acb483. [DOI] [PubMed] [Google Scholar]
- *Machado, L. F., Watanabe P. C. A., Rodrigues G. A., and Junior L. O. M.. 2023. “Deep Learning for Automatic Mandible Segmentation on Dental Panoramic X‐Ray Images.” Biomedical Physics & Engineering Express9, no. 3: 035015. 10.1088/2057-1976/acb7f6. [DOI] [PubMed] [Google Scholar]
- *Mahdi, F. P., Motoki K., and Kobashi S.. 2020. “Optimization Technique Combined With Deep Learning Method for Teeth Recognition in Dental Panoramic Radiographs.” Scientific Reports10, no. 1: 19261. 10.1038/s41598-020-75887-9. [DOI] [PMC free article] [PubMed] [Google Scholar]
- *Mao, Y.‐C., Chen T.‐Y., Chou H.‐S., et al. 2021. “Caries and Restoration Detection Using Bitewing Film Based on Transfer Learning With Cnns.” Sensors21, no. 13: 4613. 10.3390/s21134613. [DOI] [PMC free article] [PubMed] [Google Scholar]
- *Mertens, S., Krois J., Cantu A. G., Arsiwala L. T., and Schwendicke F.. 2021. “Artificial Intelligence for Caries Detection: Randomized Trial.” Journal of Dentistry115: 103849. 10.1016/j.jdent.2021.103849. [DOI] [PubMed] [Google Scholar]
- *Miki, Y., Muramatsu C., Hayashi T., et al. 2017. “Classification of Teeth in Cone‐Beam CT Using Deep Convolutional Neural Network.” Computers in Biology and Medicine80: 24–29. 10.1016/j.compbiomed.2016.11.003. [DOI] [PubMed] [Google Scholar]
- Mima, Y., Nakayama R., Hizukuri A., and Murata K.. 2022. “Tooth Detection for Each Tooth Type by Application of Faster R‐CNNs to Divided Analysis Areas of Dental Panoramic X‐Ray Images.” Radiological Physics and Technology15, no. 2: 170–176. 10.1007/s12194-022-00659-1. [DOI] [PubMed] [Google Scholar]
- *Min Park, Y., Yol Lim J., Woo Koh Y., Kim S. H., and Chang Choi E.. 2021. “Prediction of Treatment Outcome Using MRI Radiomics and Machine Learning in Oropharyngeal Cancer Patients After Surgical Treatment.” Oral Oncology122: 105559. 10.1016/j.oraloncology.2021.105559. [DOI] [PubMed] [Google Scholar]
- *Mine, Y., Iwamoto Y., Okazaki S., et al. 2022. “Detecting the Presence of Supernumerary Teeth During the Early Mixed Dentition Stage Using Deep Learning Algorithms: A Pilot Study.” International Journal of Paediatric Dentistry32, no. 5: 678–685. 10.1111/ipd.12946. [DOI] [PubMed] [Google Scholar]
- *Mohammad, N., Muad A. M., Ahmad R., and Yusof M. Y. P. M.. 2022. “Accuracy of Advanced Deep Learning With Tensorflow and Keras for Classifying Teeth Developmental Stages in Digital Panoramic Imaging.” BMC Medical Imaging22, no. 1: 66. 10.1186/s12880-022-00794-6. [DOI] [PMC free article] [PubMed] [Google Scholar]
- *Moran, M., Faria M., Giraldi G., Bastos L., Oliveira L., and Conci A.. 2021. “Classification of Approximal Caries in Bitewing Radiographs Using Convolutional Neural Networks.” Sensors21, no. 15: 5192. 10.3390/s21155192. [DOI] [PMC free article] [PubMed] [Google Scholar]
- *Mori, M., Ariji Y., Fukuda M., et al. 2022. “Performance of Deep Learning Technology for Evaluation of Positioning Quality in Periapical Radiography of the Maxillary Canine.” Oral Radiology38, no. 1: 147–154. 10.1007/s11282-021-00538-2. [DOI] [PMC free article] [PubMed] [Google Scholar]
- *Morishita, T., Muramatsu C., Seino Y., et al. 2022. “Tooth Recognition of 32 Tooth Types by Branched Single Shot Multibox Detector and Integration Processing in Panoramic Radiographs.” Journal of Medical Imaging9, no. 3: 034503. 10.1117/1.JMI.9.3.034503. [DOI] [PMC free article] [PubMed] [Google Scholar]
- *Morita, D., Mazen S., Tsujiko S., Otake Y., Sato Y., and Numajiri T.. 2023. “Deep‐Learning‐Based Automatic Facial Bone Segmentation Using a Two‐Dimensional U‐Net.” International Journal of Oral and Maxillofacial Surgery52, no. 7: 787–792. 10.1016/j.ijom.2022.10.015. [DOI] [PubMed] [Google Scholar]
- *Mortaheb, P., and Rezaeian M.. 2016. “Metal Artifact Reduction and Segmentation of Dental Computerized Tomography Images Using Least Square Support Vector Machine and Mean Shift Algorithm.” Journal of Medical Signals & Sensors6, no. 1: 1–11. 10.4103/2228-7477.175867. [DOI] [PMC free article] [PubMed] [Google Scholar]
- *Muhammed Sunnetci, K., Ulukaya S., and Alkan A.. 2022. “Periodontal Bone Loss Detection Based on Hybrid Deep Learning and Machine Learning Models With a User‐Friendly Application.” Biomedical Signal Processing and Control77: 103844. 10.1016/j.bspc.2022.103844. [DOI] [Google Scholar]
- *Muramatsu, C., Horiba K., Hayashi T., et al. 2016. “Quantitative Assessment of Mandibular Cortical Erosion on Dental Panoramic Radiographs for Screening Osteoporosis.” International Journal of Computer Assisted Radiology and Surgery11, no. 11: 2021–2032. 10.1007/s11548-016-1438-8. [DOI] [PubMed] [Google Scholar]
- *Muramatsu, C., Morishita T., Takahashi R., et al. 2021. “Tooth Detection and Classification on Panoramic Radiographs for Automatic Dental Chart Filing: Improved Classification by Multi‐Sized Input Data.” Oral Radiology37, no. 1: 13–19. 10.1007/s11282-019-00418-w. [DOI] [PubMed] [Google Scholar]
- *Murata, M., Ariji Y., Ohashi Y., et al. 2019. “Deep‐Learning Classification Using Convolutional Neural Network for Evaluation of Maxillary Sinusitis on Panoramic Radiography.” Oral Radiology35, no. 3: 301–307. 10.1007/s11282-018-0363-7. [DOI] [PubMed] [Google Scholar]
- *Nakamoto, T., Taguchi A., and Kakimoto N.. 2022. “Osteoporosis Screening Support System From Panoramic Radiographs Using Deep Learning by Convolutional Neural Network.” Dento Maxillo Facial Radiology51, no. 6: 20220135. 10.1259/dmfr.20220135. [DOI] [PMC free article] [PubMed] [Google Scholar]
- *Ngan, T. T., Tuan T. M., Son L. H., Minh N. H., and Dey N.. 2016. “Decision Making Based on Fuzzy Aggregation Operators for Medical Diagnosis From Dental X‐Ray Images.” Journal of Medical Systems40, no. 12: 280. 10.1007/s10916-016-0634-y. [DOI] [PubMed] [Google Scholar]
- *Ngoc, V. T. N., Agwu A. C., Son L. H., et al. 2020. “The Combination of Adaptive Convolutional Neural Network and Bag of Visual Words in Automatic Diagnosis of Third Molar Complications on Dental X‐Ray Images.” Diagnostics10, no. 4: 209. 10.3390/diagnostics10040209. [DOI] [PMC free article] [PubMed] [Google Scholar]
- *Nishiyama, M., Ishibashi K., Ariji Y., et al. 2021. “Performance of Deep Learning Models Constructed Using Panoramic Radiographs From Two Hospitals to Diagnose Fractures of the Mandibular Condyle.” Dento Maxillo Facial Radiology50, no. 7: 20200611. 10.1259/dmfr.20200611. [DOI] [PMC free article] [PubMed] [Google Scholar]
- *Noguchi, K., Saito I., Namiki T., Yoshimura Y., and Nakaguchi T.. 2023. “Reliability of Non‐Contact Tongue Diagnosis for Sjögren's Syndrome Using Machine Learning Method.” Scientific Reports13, no. 1: 1334. 10.1038/s41598-023-27764-4. [DOI] [PMC free article] [PubMed] [Google Scholar]
- *Okada, K., Rysavy S., Flores A., and Linguraru M. G.. 2015. “Noninvasive Differential Diagnosis of Dental Periapical Lesions in Cone‐Beam CT Scans.” Medical Physics42, no. 4: 1653–1665. 10.1118/1.4914418. [DOI] [PubMed] [Google Scholar]
- *Orhan, K., Bilgir E., Bayrakdar I. S., Ezhov M., Gusarev M., and Shumilov E.. 2021. “Evaluation of Artificial Intelligence for Detecting Impacted Third Molars on Cone‐Beam Computed Tomography Scans.” Journal of Stomatology, Oral and Maxillofacial Surgery122, no. 4: 333–337. 10.1016/j.jormas.2020.12.006. [DOI] [PubMed] [Google Scholar]
- *Oztekin, F., Katar O., Sadak F., et al. 2023. “An Explainable Deep Learning Model to Prediction Dental Caries Using Panoramic Radiograph Images.” Diagnostics13, no. 2: 226. 10.3390/diagnostics13020226. [DOI] [PMC free article] [PubMed] [Google Scholar]
- *Paderno, A., Piazza C., Del Bon F., et al. 2021. “Deep Learning for Automatic Segmentation of Oral and Oropharyngeal Cancer Using Narrow Band Imaging: Preliminary Experience in a Clinical Perspective.” Frontiers in Oncology11: 626602. 10.3389/fonc.2021.626602. [DOI] [PMC free article] [PubMed] [Google Scholar]
- *Pan, X., Zhang T., Yang Q., Yang D., Rwigema J. C., and Qi X. S.. 2020. “Survival Prediction for Oral Tongue Cancer Patients via Probabilistic Genetic Algorithm Optimized Neural Network Models.” British Journal of Radiology93, no. 1112: 20190825. 10.1259/bjr.20190825. [DOI] [PMC free article] [PubMed] [Google Scholar]
- *Park, E. Y., Cho H., Kang S., Jeong S., and Kim E.‐K.. 2022. “Caries Detection With Tooth Surface Segmentation on Intraoral Photographic Images Using Deep Learning.” BMC Oral Health22, no. 1: 573. 10.1186/s12903-022-02589-1. [DOI] [PMC free article] [PubMed] [Google Scholar]
- *Park, H. S., Seo J. K., Hyun C. M., Lee S. M., and Jeon K.. 2022. “A Fidelity‐Embedded Learning for Metal Artifact Reduction in Dental CBCT.” Medical Physics49, no. 8: 5195–5205. 10.1002/mp.15720. [DOI] [PubMed] [Google Scholar]
- *Prados‐Privado, M., García Villalón J., Blázquez Torres A., Martínez‐Martínez C. H., and Ivorra C.. 2021. “A Convolutional Neural Network for Automatic Tooth Numbering in Panoramic Images.” BioMed Research International2021: 1–7. 10.1155/2021/3625386. [DOI] [PMC free article] [PubMed] [Google Scholar]
- *Prados‐Privado, M., Villalón J. G., Torres A. B., Martínez‐Martínez C. H., and Ivorra C.. 2021a. “A Validation Employing Convolutional Neural Network for the Radiographic Detection of Absence or Presence of Teeth.” Journal of Clinical Medicine10, no. 6: 1–12. 10.3390/jcm10061186. [DOI] [PMC free article] [PubMed] [Google Scholar]
- *Preda, F., Morgan N., Van Gerven A., et al. 2022. “Deep Convolutional Neural Network‐Based Automated Segmentation of the Maxillofacial Complex From Cone‐Beam Computed Tomography: A Validation Study.” Journal of Dentistry124: 104238. 10.1016/j.jdent.2022.104238. [DOI] [PubMed] [Google Scholar]
- *Qayyum, A., Tahir A., Butt M. A., et al. 2023. “Dental Caries Detection Using a Semi‐Supervised Learning Approach.” Scientific Reports13, no. 1: 749. 10.1038/s41598-023-27808-9. [DOI] [PMC free article] [PubMed] [Google Scholar]
- *Qiu, B., van derWel H., Kraeima J., et al. 2021. “Robust and Accurate Mandible Segmentation on Dental CBCT Scans Affected by Metal Artifacts Using a Prior Shape Model.” Journal of Personalized Medicine11, no. 5: 364. 10.3390/jpm11050364. [DOI] [PMC free article] [PubMed] [Google Scholar]
- *Qiu, B., Guo J., Kraeima J., et al. 2019. “Automatic Segmentation of the Mandible From Computed Tomography Scans for 3D Virtual Surgical Planning Using the Convolutional Neural Network.” Physics in Medicine & Biology64, no. 17: 175020. 10.1088/1361-6560/ab2c95. [DOI] [PubMed] [Google Scholar]
- *Qu, Y., Lin Z., Yang Z., Lin H., Huang X., and Gu L.. 2022. “Machine Learning Models for Prognosis Prediction in Endodontic Microsurgery.” Journal of Dentistry118: 103947. 10.1016/j.jdent.2022.103947. [DOI] [PubMed] [Google Scholar]
- *Raith, S., Vogel E. P., Anees N., et al. 2017. “Artificial Neural Networks as a Powerful Numerical Tool to Classify Specific Features of a Tooth Based on 3D Scan Data.” Computers in Biology and Medicine80: 65–76. 10.1016/j.compbiomed.2016.11.013. [DOI] [PubMed] [Google Scholar]
- *Romeo, V., Cuocolo R., Ricciardi C., et al. 2020. “Prediction of Tumor Grade and Nodal Status in Oropharyngeal and Oral Cavity Squamous‐Cell Carcinoma Using a Radiomic Approach.” Anticancer Research40, no. 1: 271–280. 10.21873/anticanres.13949. [DOI] [PubMed] [Google Scholar]
- *Ryu, J., Kim Y.‐H., Kim T.‐W., and Jung S.‐K.. 2023. “Evaluation of Artificial Intelligence Model for Crowding Categorization and Extraction Diagnosis Using Intraoral Photographs.” Scientific Reports13, no. 1: 5177. 10.1038/s41598-023-32514-7. [DOI] [PMC free article] [PubMed] [Google Scholar]
- *Schlickenrieder, A., Meyer O., Schönewolf J., et al. 2021. “Automatized Detection and Categorization of Fissure Sealants From Intraoral Digital Photographs Using Artificial Intelligence.” Diagnostics11, no. 9: 1608. 10.3390/diagnostics11091608. [DOI] [PMC free article] [PubMed] [Google Scholar]
- *Schönewolf, J., Meyer O., Engels P., et al. 2022. “Artificial Intelligence‐Based Diagnostics of Molar‐Incisor‐Hypomineralization (MIH) on Intraoral Photographs.” Clinical Oral Investigations26, no. 9: 5923–5930. 10.1007/s00784-022-04552-4. [DOI] [PMC free article] [PubMed] [Google Scholar]
- Schwendicke, F., Mertens S., Cantu A. G., Chaurasia A., Meyer‐Lueckel H., and Krois J.. 2022. “Cost‐Effectiveness of AI for Caries Detection: Randomized Trial.” J Dent119: 104080. 10.1016/j.jdent.2022.104080. [DOI] [PubMed] [Google Scholar]
- Seo, H., Badiei Khuzani M., Vasudevan V., et al. 2020. “Machine Learning Techniques for Biomedical Image Segmentation: An Overview of Technical Aspects and Introduction to State‐of‐Art Applications.” Medical Physics47, no. 5: e148–e167. 10.1002/mp.13649. [DOI] [PMC free article] [PubMed] [Google Scholar]
- *Seo, H., Hwang J., Jeong T., and Shin J.. 2021. “Comparison of Deep Learning Models for Cervical Vertebral Maturation Stage Classification on Lateral Cephalometric Radiographs.” Journal of Clinical Medicine10, no. 16: 3591. 10.3390/jcm10163591. [DOI] [PMC free article] [PubMed] [Google Scholar]
- *Shahnavazi, M., and Mohamadrahimi H.. 2023. “The Application of Artificial Neural Networks in the Detection of Mandibular Fractures Using Panoramic Radiography.” Dental Research Journal20, no. 1: 27. 10.4103/1735-3327.369629. [DOI] [PMC free article] [PubMed] [Google Scholar]
- *Shin, W., Yeom H.‐G., Lee G. H., et al. 2021. “Deep Learning Based Prediction of Necessity for Orthognathic Surgery of Skeletal Malocclusion Using Cephalogram in Korean Individuals.” BMC Oral Health21, no. 1: 130. 10.1186/s12903-021-01513-3. [DOI] [PMC free article] [PubMed] [Google Scholar]
- Singh, Y., Atulkar V., Ren J., et al. 2021. Osteoporosis Prescreening and Bone Mineral Density Prediction using Dental Panoramic Radiographs. Institute of Electrical and Electronics Engineers Inc. 10.1109/EMBC46164.2021.9630183. [DOI] [PubMed] [Google Scholar]
- *Son, D.‐M., Yoon Y.‐A., Kwon H.‐J., An C.‐H., and Lee S.‐H.. 2021. “Automatic Detection of Mandibular Fractures in Panoramic Radiographs Using Deep Learning.” Diagnostics11, no. 6: 933. 10.3390/diagnostics11060933. [DOI] [PMC free article] [PubMed] [Google Scholar]
- *Song, B., Li S., Sunny S., et al. 2022. “Exploring Uncertainty Measures in Convolutional Neural Network for Semantic Segmentation of Oral Cancer Images.” Journal of Biomedical Optics27, no. 11: 115001. 10.1117/1.JBO.27.11.115001. [DOI] [PMC free article] [PubMed] [Google Scholar]
- *Song, B., Sunny S., Uthoff R. D., et al. 2018. “Automatic Classification of Dual‐Modalilty, Smartphone‐Based Oral Dysplasia and Malignancy Images Using Deep Learning.” Biomedical Optics Express9, no. 11: 5318–5329. 10.1364/BOE.9.005318. [DOI] [PMC free article] [PubMed] [Google Scholar]
- *Song, B., Zhang C., Sunny S., et al. 2023. “Interpretable and Reliable Oral Cancer Classifier With Attention Mechanism and Expert Knowledge Embedding via Attention Map.” Cancers15, no. 5: 1421. 10.3390/cancers15051421. [DOI] [PMC free article] [PubMed] [Google Scholar]
- *Sukegawa, S., Fujimura A., Taguchi A., et al. 2022. “Identification of Osteoporosis Using Ensemble Deep Learning Model With Panoramic Radiographs and Clinical Covariates.” Scientific Reports12, no. 1: 6088. 10.1038/s41598-022-10150-x. [DOI] [PMC free article] [PubMed] [Google Scholar]
- *Sukegawa, S., Matsuyama T., Tanaka F., et al. 2022a. “Evaluation of Multi‐Task Learning in Deep Learning‐Based Positioning Classification of Mandibular Third Molars.” Scientific Reports12, no. 1. 10.1038/s41598-021-04603-y. [DOI] [PMC free article] [PubMed] [Google Scholar]
- *Sukegawa, S., Yoshii K., Hara T., et al. 2020. “Deep Neural Networks for Dental Implant System Classification.” Biomolecules10, no. 7: 984. 10.3390/biom10070984. [DOI] [PMC free article] [PubMed] [Google Scholar]
- *Sukegawa, S., Yoshii K., Hara T., et al. 2022b. “Is Attention Branch Network Effective in Classifying Dental Implants From Panoramic Radiograph Images by Deep Learning?” PLoS One17, no. 7: e0269016. 10.1371/journal.pone.0269016. [DOI] [PMC free article] [PubMed] [Google Scholar]
- *Sun, T.‐G., Mao L., Chai Z.‐K., Shen X.‐M., and Sun Z.‐J.. 2022. “Predicting the Proliferation of Tongue Cancer With Artificial Intelligence in Contrast‐Enhanced CT.” Frontiers in Oncology12: 841262. 10.3389/fonc.2022.841262. [DOI] [PMC free article] [PubMed] [Google Scholar]
- *Takahashi, T., Nozaki K., Gonda T., and Ikebe K.. 2021. “A System for Designing Removable Partial Dentures Using Artificial Intelligence. Part 1. Classification of Partially Edentulous Arches Using a Convolutional Neural Network.” Journal of Prosthodontic Research65, no. 1: 115–118. 10.2186/jpr.JPOR_2019_354. [DOI] [PubMed] [Google Scholar]
- *Takebe, K., Imai T., Kubota S., Nishimoto A., Amekawa S., and Uzawa N.. 2023. “Deep Learning Model for the Automated Evaluation of Contact Between the Lower Third Molar and Inferior Alveolar Nerve on Panoramic Radiography.” Journal of Dental Sciences18: 991–996. 10.1016/j.jds.2022.12.008. [DOI] [PMC free article] [PubMed] [Google Scholar]
- *Tao, S., and Wang Z.. 2022. “Tooth CT Image Segmentation Method Based on the U‐Net Network and Attention Module.” Computational and Mathematical Methods in Medicine2022: 1–8. 10.1155/2022/3289663. [DOI] [PMC free article] [PubMed] [Google Scholar]
- *Tareq, A., Faisal M. I., Islam M. S., et al. 2023. “Visual Diagnostics of Dental Caries Through Deep Learning of Non‐Standardised Photographs Using a Hybrid YOLO Ensemble and Transfer Learning Model.” International Journal of Environmental Research and Public Health20, no. 7: 5351. 10.3390/ijerph20075351. [DOI] [PMC free article] [PubMed] [Google Scholar]
- *Tian, S., Huang R., Li Z., et al. 2022. “A Dual Discriminator Adversarial Learning Approach for Dental Occlusal Surface Reconstruction.” Journal of Healthcare Engineering2022: 1–14. 10.1155/2022/1933617. [DOI] [PMC free article] [PubMed] [Google Scholar]
- *De Tobel, J., Radesh P., Vandermeulen D., and Thevissen P. W.. 2017. “An Automated Technique to Stage Lower Third Molar Development on Panoramic Radiographs for Age Estimation: A Pilot Study.” Journal of Forensic Odonto‐Stomatology2, no. 35: 49–60. [PMC free article] [PubMed] [Google Scholar]
- *Tobias, M. A. S., Nogueira B. P., Santana M. C. S., Pires R. G., Papa J. P., and Santos P. S. S.. 2022. “Artificial Intelligence for Oral Cancer Diagnosis: What Are the Possibilities?” Oral Oncology134: 106117. 10.1016/j.oraloncology.2022.106117. [DOI] [PubMed] [Google Scholar]
- *Tomita, H., Yamashiro T., Heianna J., et al. 2021. “Nodal‐Based Radiomics Analysis for Identifying Cervical Lymph Node Metastasis at Levels I and II in Patients With Oral Squamous Cell Carcinoma Using Contrast‐Enhanced Computed Tomography.” European Radiology31, no. 10: 7440–7449. 10.1007/s00330-021-07758-4. [DOI] [PubMed] [Google Scholar]
- *Tomita, H., Yamashiro T., Heianna J., et al. 2021a. “Deep Learning for the Preoperative Diagnosis of Metastatic Cervical Lymph Nodes on Contrast‐Enhanced Computed Tomography in Patients With Oral Squamous Cell Carcinoma.” Cancers13, no. 4: 1–11. 10.3390/cancers13040600. [DOI] [PMC free article] [PubMed] [Google Scholar]
- Tricco, A. C., Lillie E., Zarin W., et al. 2018. “Prisma Extension for Scoping Reviews (PRISMA‐SCR): Checklist and Explanation.” Annals of Internal Medicine169, no. 7: 467–473. 10.7326/m18-0850. [DOI] [PubMed] [Google Scholar]
- *Verhelst, P. J., Smolders A., Beznik T., et al. 2021. “Layered Deep Learning for Automatic Mandibular Segmentation in Cone‐Beam Computed Tomography.” Journal of Dentistry114: 103786. 10.1016/j.jdent.2021.103786. [DOI] [PubMed] [Google Scholar]
- *Vila‐Blanco, N., Varas‐Quintana P., Aneiros‐Ardao Á., Tomás I., and Carreira M. J.. 2021. “Automated Description of the Mandible Shape by Deep Learning.” International Journal of Computer Assisted Radiology and Surgery16, no. 12: 2215–2224. 10.1007/s11548-021-02474-2. [DOI] [PMC free article] [PubMed] [Google Scholar]
- *Vinayahalingam, S., Berends B., Baan F., et al. 2023. “Deep Learning for Automated Segmentation of the Temporomandibular Joint.” Journal of Dentistry132: 104475. 10.1016/j.jdent.2023.104475. [DOI] [PubMed] [Google Scholar]
- *Vinayahalingam, S., Kempers S., Limon L., et al. 2021. “Classification of Caries in Third Molars on Panoramic Radiographs Using Deep Learning.” Scientific Reports11, no. 1: 12609. 10.1038/s41598-021-92121-2. [DOI] [PMC free article] [PubMed] [Google Scholar]
- *Vinayahalingam, S., van Nistelrooij N., van Ginneken B., et al. 2022. “Detection of Mandibular Fractures on Panoramic Radiographs Using Deep Learning.” Scientific Reports12, no. 1: 19596. 10.1038/s41598-022-23445-w. [DOI] [PMC free article] [PubMed] [Google Scholar]
- *Vollmer, A., Saravi B., Vollmer M., et al. 2022. “Artificial Intelligence‐Based Prediction of Oroantral Communication After Tooth Extraction Utilizing Preoperative Panoramic Radiography.” Diagnostics12, no. 6: 1406. 10.3390/diagnostics12061406. [DOI] [PMC free article] [PubMed] [Google Scholar]
- *Vranckx, M., Van Gerven A., Willems H., et al. 2020. “Artificial Intelligence (AI)‐Driven Molar Angulation Measurements to Predict Third Molar Eruption on Panoramic Radiographs.” International Journal of Environmental Research and Public Health17, no. 10: 3716. 10.3390/ijerph17103716. [DOI] [PMC free article] [PubMed] [Google Scholar]
- *Wang, C., Qin H., Lai G., et al. 2020. “Automated Classification of Dual Channel Dental Imaging of Auto‐Fluorescence and White Lightby Convolutional Neural Networks.” Journal of Innovative Optical Health Sciences13, no. 4: 2050014. 10.1142/S1793545820500145. [DOI] [Google Scholar]
- *Wang, C., Zhang R., Wei X., Wang L., Wu P., and Yao Q.. 2023. “Deep Learning and Sub‐Band Fluorescence Imaging‐Based Method for Caries and Calculus Diagnosis Embeddable on Different Smartphones.” Biomedical Optics Express14, no. 2: 866–882. 10.1364/BOE.479818. [DOI] [PMC free article] [PubMed] [Google Scholar]
- *Wang, X., Meng X., and Yan S.. 2021. “Deep Learning‐Based Image Segmentation of Cone‐Beam Computed Tomography Images for Oral Lesion Detection.” Journal of Healthcare Engineering2021: 4603475. 10.1155/2021/4603475. [DOI] [PMC free article] [PubMed] [Google Scholar]
- *Wang, X., Xu Z., Tong Y., et al. 2022. “Detection and Classification of Mandibular Fracture on CT Scan Using Deep Convolutional Neural Network.” Clinical Oral Investigations26, no. 6: 4593–4601. 10.1007/s00784-022-04427-8. [DOI] [PubMed] [Google Scholar]
- *Wang, X., Zhao X., Song G., Niu J., and Xu T.. 2022a. “Machine Learning‐Based Evaluation on Craniodentofacial Morphological Harmony of Patients After Orthodontic Treatment.” Frontiers in Physiology13: 862847. 10.3389/fphys.2022.862847. [DOI] [PMC free article] [PubMed] [Google Scholar]
- *Warin, K., Limprasert W., Suebnukarn S., Inglam S., Jantana P., and Vicharueang S.. 2022. “Assessment of Deep Convolutional Neural Network Models for Mandibular Fracture Detection in Panoramic Radiographs.” International Journal of Oral and Maxillofacial Surgery51, no. 11: 1488–1494. 10.1016/j.ijom.2022.03.056. [DOI] [PubMed] [Google Scholar]
- *Warin, K., Limprasert W., Suebnukarn S., Jinaporntham S., and Jantana P.. 2021. “Automatic Classification and Detection of Oral Cancer in Photographic Images Using Deep Learning Algorithms.” Journal of Oral Pathology & Medicine50, no. 9: 911–918. 10.1111/jop.13227. [DOI] [PubMed] [Google Scholar]
- *Warin, K., Limprasert W., Suebnukarn S., Jinaporntham S., and Jantana P.. 2022a. “Performance of Deep Convolutional Neural Network for Classification and Detection of Oral Potentially Malignant Disorders in Photographic Images.” International Journal of Oral and Maxillofacial Surgery51, no. 5: 699–704. 10.1016/j.ijom.2021.09.001. [DOI] [PubMed] [Google Scholar]
- *Warin, K., Limprasert W., Suebnukarn S., Jinaporntham S., Jantana P., and Vicharueang S.. 2022b. “AI‐Based Analysis of Oral Lesions Using Novel Deep Convolutional Neural Networks for Early Detection of Oral Cancer.” PLoS One17, no. 8: e0273508. 10.1371/journal.pone.0273508. [DOI] [PMC free article] [PubMed] [Google Scholar]
- *Watanabe, H., Ariji Y., Fukuda M., et al. 2021. “Deep Learning Object Detection of Maxillary Cyst‐Like Lesions on Panoramic Radiographs: Preliminary Study.” Oral Radiology37, no. 3: 487–493. 10.1007/s11282-020-00485-4. [DOI] [PubMed] [Google Scholar]
- *Welch, M. L., McIntosh C., Purdie T. G., et al. 2020. “Automatic Classification of Dental Artifact Status for Efficient Image Veracity Checks: Effects of Image Resolution and Convolutional Neural Network Depth.” Physics in Medicine & Biology65, no. 1: 015005. 10.1088/1361-6560/ab5427. [DOI] [PubMed] [Google Scholar]
- *Welch, M. L., McIntosh C., Traverso A., et al. 2020. “External Validation and Transfer Learning of Convolutional Neural Networks for Computed Tomography Dental Artifact Classification.” Physics in Medicine & Biology65, no. 3: 035017. 10.1088/1361-6560/ab63ba. [DOI] [PubMed] [Google Scholar]
- *Widyaningrum, R., Sela E. I., Pulungan R., and Septiarini A.. 2023. “Automatic Segmentation of Periapical Radiograph Using Color Histogram and Machine Learning for Osteoporosis Detection.” International Journal of Dentistry2023: 1–9. 10.1155/2023/6662911. [DOI] [PMC free article] [PubMed] [Google Scholar]
- *Wu, C., Chang F., Su X., et al. 2022. “Integrating Features From Lymph Node Stations for Metastatic Lymph Node Detection.” Computerized Medical Imaging and Graphics101: 102108. 10.1016/j.compmedimag.2022.102108. [DOI] [PubMed] [Google Scholar]
- *Wu, T.‐H., Lian C., Lee S., et al. 2022. “Two‐Stage Mesh Deep Learning for Automated Tooth Segmentation and Landmark Localization on 3D Intraoral Scans.” IEEE Transactions on Medical Imaging41, no. 11: 3158–3166. 10.1109/TMI.2022.3180343. [DOI] [PMC free article] [PubMed] [Google Scholar]
- *Xia, Z., Gan Y., Chang L., Xiong J., and Zhao Q.. 2017. “Individual Tooth Segmentation From Ct Images Scanned With Contacts of Maxillary and Mandible Teeth.” Computer Methods and Programs in Biomedicine138: 1–12. 10.1016/j.cmpb.2016.10.002. [DOI] [PubMed] [Google Scholar]
- *Xiao, Y., Liang Q., Zhou L., et al. 2022. “Construction of a New Automatic Grading System for Jaw Bone Mineral Density Level Based on Deep Learning Using Cone Beam Computed Tomography.” Scientific Reports12, no. 1: 12841. 10.1038/s41598-022-16074-w. [DOI] [PMC free article] [PubMed] [Google Scholar]
- *Xie, L., Tang W., Izadikhah I., et al. 2022. “Intelligent Quantitative Assessment of Skeletal Maturation Based on Multi‐Stage Model: A Retrospective Cone‐Beam CT Study of Cervical Vertebrae.” Oral Radiology38, no. 3: 378–388. 10.1007/s11282-021-00566-y. [DOI] [PubMed] [Google Scholar]
- *Xu, J., Liu J., Zhang D., et al. 2021. “Automatic Mandible Segmentation from CT Image Using 3D Fully Convolutional Neural Network Based on DenseASPP and Attention Gates.” International Journal of Computer Assisted Radiology and Surgery16, no. 10: 1785–1794. 10.1007/s11548-021-02447-5. [DOI] [PubMed] [Google Scholar]
- *Xu, J., Liu J., Zhang D., Zhou Z., Zhang C., and Chen X.. 2021a. “A 3D Segmentation Network of Mandible From CT Scan With Combination of Multiple Convolutional Modules and Edge Supervision in Mandibular Reconstruction.” Computers in Biology and Medicine138: 104925. 10.1016/j.compbiomed.2021.104925. [DOI] [PubMed] [Google Scholar]
- Xu, X., Liu C., and Zheng Y.. 2019. “3D Tooth Segmentation and Labeling Using Deep Convolutional Neural Networks.” IEEE Transactions on Visualization and Computer Graphics25, no. 7: 2336–2348. 10.1109/TVCG.2018.2839685. [DOI] [PubMed] [Google Scholar]
- *Xu, X., Xi L., Wei L., et al. 2023. “Deep Learning Assisted Contrast‐Enhanced Ct–Based Diagnosis of Cervical Lymph Node Metastasis of Oral Cancer: A Retrospective Study of 1466 Cases.” European Radiology33, no. 6: 4303–4312. 10.1007/s00330-022-09355-5. [DOI] [PMC free article] [PubMed] [Google Scholar]
- *Yadollahi, M., Procházka A., Kašparová M., Vyšata O., and Mařík V.. 2015. “Separation of Overlapping Dental Arch Objects Using Digital Records of Illuminated Plaster Casts.” BioMedical Engineering Online14, no. 1: 67. 10.1186/s12938-015-0066-9. [DOI] [PMC free article] [PubMed] [Google Scholar]
- *Yang, H., Jo E., Kim H. J., et al. 2020. “Deep Learning for Automated Detection of Cyst and Tumors of the Jaw in Panoramic Radiographs.” Journal of Clinical Medicine9, no. 6: 1839. 10.3390/jcm9061839. [DOI] [PMC free article] [PubMed] [Google Scholar]
- *Yang, P., Guo X., Mu C., Qi S., and Li G.. 2023. “Detection of Vertical Root Fractures by Cone‐Beam Computed Tomography Based on Deep Learning.” Dentomaxillofacial Radiology52, no. 3: 20220345. 10.1259/dmfr.20220345. [DOI] [PMC free article] [PubMed] [Google Scholar]
- *Yang, S., Lee H., Jang B., et al. 2022. “Development and Validation of a Visually Explainable Deep Learning Model for Classification of C‐Shaped Canals of the Mandibular Second Molars in Periapical and Panoramic Dental Radiographs.” Journal of Endodontics48, no. 7: 914–921. 10.1016/j.joen.2022.04.007. [DOI] [PubMed] [Google Scholar]
- *Yao, J., Zeng W., He T., et al. 2022. “Automatic Localization of Cephalometric Landmarks Based on Convolutional Neural Network.” American Journal of Orthodontics and Dentofacial Orthopedics161, no. 3: e250–e259. 10.1016/j.ajodo.2021.09.012. [DOI] [PubMed] [Google Scholar]
- *Yaren Tekin, B., Ozcan C., Pekince A., and Yasa Y.. 2022. “An Enhanced Tooth Segmentation and Numbering According to FDI Notation in Bitewing Radiographs.” Computers in Biology and Medicine146: 105547. 10.1016/j.compbiomed.2022.105547. [DOI] [PubMed] [Google Scholar]
- *Yesiltepe, S., Bayrakdar I. S., Orhan K., et al. 2022. “A Deep Learning Model for Idiopathic Osteosclerosis Detection on Panoramic Radiographs.” Medical Principles and Practice31, no. 6: 555–561. 10.1159/000527145. [DOI] [PMC free article] [PubMed] [Google Scholar]
- *You, W., Hao A., Li S., Wang Y., and Xia B.. 2020. “Deep Learning‐Based Dental Plaque Detection on Primary Teeth: A Comparison With Clinical Assessments.” BMC Oral Health20, no. 1: 141. 10.1186/s12903-020-01114-6. [DOI] [PMC free article] [PubMed] [Google Scholar]
- *Yu, D., Hu J., Feng Z., Song M., and Zhu H.. 2022. “Deep Learning Based Diagnosis for Cysts and Tumors of Jaw With Massive Healthy Samples.” Scientific Reports12, no. 1: 1855. 10.1038/s41598-022-05913-5. [DOI] [PMC free article] [PubMed] [Google Scholar]
- *Yüksel, A. E., Gültekin S., Simsar E., et al. 2021. “Dental Enumeration and Multiple Treatment Detection on Panoramic X‐Rays Using Deep Learning.” Scientific Reports11, no. 1: 12342. 10.1038/s41598-021-90386-1. [DOI] [PMC free article] [PubMed] [Google Scholar]
- *Zadrożny, Ł., Regulski P., Brus‐Sawczuk K., et al. 2022. “Artificial Intelligence Application in Assessment of Panoramic Radiographs.” Diagnostics12, no. 1: 224. 10.3390/diagnostics12010224. [DOI] [PMC free article] [PubMed] [Google Scholar]
- *Zhang, C., Fan L., Zhang S., Zhao J., and Gu Y.. 2023. “Deep Learning Based Dental Implant Failure Prediction From Periapical and Panoramic Films.” Quantitative Imaging in Medicine and Surgery13, no. 2: 935–945. 10.21037/qims-22-457. [DOI] [PMC free article] [PubMed] [Google Scholar]
- *Zhang, J.‐N., Lu H.‐P., Hou J., et al. 2023. “Deep Learning‐Based Prediction of Mandibular Growth Trend in Children With Anterior Crossbite Using Cephalometric Radiographs.” BMC Oral Health23, no. 1: 28. 10.1186/s12903-023-02734-4. [DOI] [PMC free article] [PubMed] [Google Scholar]
- *Zhang, X., Liang Y., Li W., et al. 2022. “Development and Evaluation of Deep Learning for Screening Dental Caries From Oral Photographs.” Oral Diseases28, no. 1: 173–181. 10.1111/odi.13735. [DOI] [PubMed] [Google Scholar]
- *Zhao, T., Zhou J., Yan J., et al. 2021. “Automated Adenoid Hypertrophy Assessment With Lateral Cephalometry in Children Based on Artificial Intelligence.” Diagnostics11, no. 8: 1386. 10.3390/diagnostics11081386. [DOI] [PMC free article] [PubMed] [Google Scholar]
- *Zhong, Y. W., Jiang Y., Dong S., et al. 2022. “Tumor Radiomics Signature for Artificial Neural Network‐Assisted Detection of Neck Metastasis in Patient With Tongue Cancer.” Journal of Neuroradiology49, no. 2: 213–218. 10.1016/j.neurad.2021.07.006. [DOI] [PubMed] [Google Scholar]
- *Zhou, X., Yu G., Yin Q., Liu Y., Zhang Z., and Sun J.. 2022. “Context Aware Convolutional Neural Network for Children Caries Diagnosis on Dental Panoramic Radiographs.” Computational and Mathematical Methods in Medicine2022: 1–8. 10.1155/2022/6029245. [DOI] [PMC free article] [PubMed] [Google Scholar]
- *Zhu, H., Yu H., Zhang F., Cao Z., Wu F., and Zhu F.. 2022. “Automatic Segmentation and Detection of Ectopic Eruption of First Permanent Molars on Panoramic Radiographs Based on nnU‐Net.” International Journal of Paediatric Dentistry32, no. 6: 785–792. 10.1111/ipd.12964. [DOI] [PubMed] [Google Scholar]
- *Zhu, T., Chen D., Wu F., Zhu F., and Zhu H.. 2021. “Artificial Intelligence Model to Detect Real Contact Relationship Between Mandibular Third Molars and Inferior Alveolar Nerve Based on Panoramic Radiographs.” Diagnostics11, no. 9: 1664. 10.3390/DIAGNOSTICS11091664. [DOI] [PMC free article] [PubMed] [Google Scholar]
Associated Data
This section collects any data citations, data availability statements, or supplementary materials included in this article.
Supplementary Materials
Supporting information.
CRE2-10-e70035-s001.docx (358KB, docx)